A REVIEW OF IMAGE DENOISING ALGORITHMS, WITH A NEW ONE
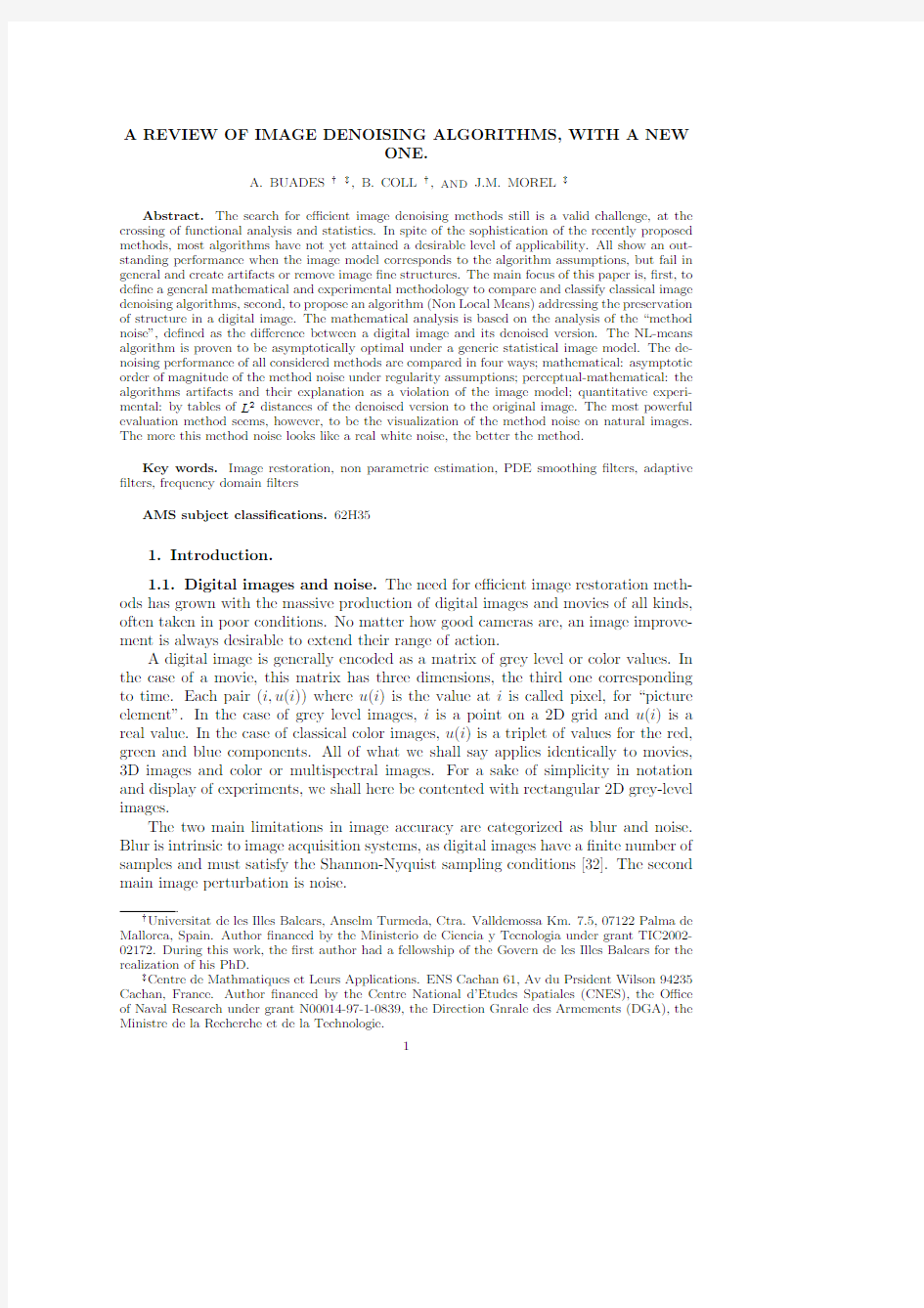

A REVIEW OF IMAGE DENOISING ALGORITHMS,WITH A NEW
ONE.
A.BUADES??,
B.COLL?,AND J.M.MOREL?
Abstract.The search for e?cient image denoising methods still is a valid challenge,at the crossing of functional analysis and statistics.In spite of the sophistication of the recently proposed methods,most algorithms have not yet attained a desirable level of applicability.All show an out-standing performance when the image model corresponds to the algorithm assumptions,but fail in general and create artifacts or remove image?ne structures.The main focus of this paper is,?rst,to de?ne a general mathematical and experimental methodology to compare and classify classical image denoising algorithms,second,to propose an algorithm(Non Local Means)addressing the preservation of structure in a digital image.The mathematical analysis is based on the analysis of the“method noise”,de?ned as the di?erence between a digital image and its denoised version.The NL-means algorithm is proven to be asymptotically optimal under a generic statistical image model.The de-noising performance of all considered methods are compared in four ways;mathematical:asymptotic order of magnitude of the method noise under regularity assumptions;perceptual-mathematical:the algorithms artifacts and their explanation as a violation of the image model;quantitative experi-mental:by tables of L2distances of the denoised version to the original image.The most powerful evaluation method seems,however,to be the visualization of the method noise on natural images. The more this method noise looks like a real white noise,the better the method.
Key words.Image restoration,non parametric estimation,PDE smoothing?lters,adaptive ?lters,frequency domain?lters
AMS subject classi?cations.62H35
1.Introduction.
1.1.Digital images and noise.The need for e?cient image restoration meth-ods has grown with the massive production of digital images and movies of all kinds, often taken in poor conditions.No matter how good cameras are,an image improve-ment is always desirable to extend their range of action.
A digital image is generally encoded as a matrix of grey level or color values.In the case of a movie,this matrix has three dimensions,the third one corresponding to time.Each pair(i,u(i))where u(i)is the value at i is called pixel,for“picture element”.In the case of grey level images,i is a point on a2D grid and u(i)is a real value.In the case of classical color images,u(i)is a triplet of values for the red, green and blue components.All of what we shall say applies identically to movies, 3D images and color or multispectral images.For a sake of simplicity in notation and display of experiments,we shall here be contented with rectangular2D grey-level images.
The two main limitations in image accuracy are categorized as blur and noise. Blur is intrinsic to image acquisition systems,as digital images have a?nite number of samples and must satisfy the Shannon-Nyquist sampling conditions[32].The second main image perturbation is noise.
?Universitat de les Illes Balears,Anselm Turmeda,Ctra.Valldemossa Km.7.5,07122Palma de Mallorca,Spain.Author?nanced by the Ministerio de Ciencia y Tecnologia under grant TIC2002-02172.During this work,the?rst author had a fellowship of the Govern de les Illes Balears for the realization of his PhD.
?Centre de Mathmatiques et Leurs Applications.ENS Cachan61,Av du Prsident Wilson94235 Cachan,France.Author?nanced by the Centre National d’Etudes Spatiales(CNES),the O?ce of Naval Research under grant N00014-97-1-0839,the Direction Gnrale des Armements(DGA),the Ministre de la Recherche et de la Technologie.
1
2 A.BUADES,B.COLL AND J.M MOREL
Each one of the pixel values u(i)is the result of a light intensity measurement, usually made by a CCD matrix coupled with a light focusing system.Each captor of the CCD is roughly a square in which the number of incoming photons is being counted for a?xed period corresponding to the obturation time.When the light source is constant,the number of photons received by each pixel?uctuates around its average in accordance with the central limit theorem.In other terms one can expect?uctuations of order
√n for n incoming photons.In addition,each captor,if not adequately cooled,receives heat spurious photons.The resulting perturbation is usually called“obscurity noise”.In a?rst rough approximation one can write
v(i)=u(i)+n(i),
where i∈I,v(i)is the observed value,u(i)would be the“true”value at pixel i, namely the one which would be observed by averaging the photon counting on a long period of time,and n(i)is the noise perturbation.As indicated,the amount of noise is signal-dependent,that is n(i)is larger when u(i)is larger.In noise models,the normalized values of n(i)and n(j)at di?erent pixels are assumed to be independent random variables and one talks about“white noise”.
1.2.Signal and noise ratios.A good quality photograph(for visual inspec-tion)has about256grey level values,where0represents black and255represents white.Measuring the amount of noise by its standard deviation,σ(n),one can de?ne the signal noise ratio(SNR)as
SNR=σ(u)σ(n)
,
whereσ(u)denotes the empirical standard deviation of u,
σ(u)= 1|I| i∈I(u(i)?u)2 12
and u=1
|I| i∈I u(i)is the average grey level value.The standard deviation of the noise can also be obtained as an empirical measurement or formally computed when
the noise model and parameters are known.A good quality image has a standard
deviation of about60.
The best way to test the e?ect of noise on a standard digital image is to add
a gaussian white noise,in which case n(i)are i.i.d.gaussian real variables.When
σ(n)=3,no visible alteration is usually observed.Thus,a60
3?20signal to noise ratio is nearly invisible.Surprisingly enough,one can add white noise up to a2
1 ratio and still see everything in a picture!This fact is illustrated in Figure1.1
and constitutes a major enigma of human vision.It justi?es the many attempts to
de?ne convincing denoising algorithms.As we shall see,the results have been rather
deceptive.Denoising algorithms see no di?erence between small details and noise,and
therefore remove them.In many cases,they create new distortions and the researchers
are so much used to them as to have created a taxonomy of denoising artifacts:
“ringing”,“blur”,“staircase e?ect”,“checkerboard e?ect”,“wavelet outliers”,etc.
This fact is not quite a surprise.Indeed,to the best of our knowledge,all denoising
algorithms are based on
?a noise model
?a generic image smoothness model,local or global.
A REVIEW OF IMAGE DENOISING ALGORIHTMS WITH A NEW ONE3
Fig.1.1.A digital image with standard deviation55,the same with noise added(standard deviation3),the signal noise ratio being therefore equal to18,and the same with signal noise ratio slightly larger than2.In this second image,no alteration is visible.In the third,a conspicuous noise with standard deviation25has been added but,surprisingly enough,all details of the original image still are visible.
In experimental settings,the noise model is perfectly precise.So the weak point of the algorithms is the inadequacy of the image model.All of the methods assume that the noise is oscillatory,and that the image is smooth,or piecewise smooth.So they try to separate the smooth or patchy part(the image)from the oscillatory one.Actually, many?ne structures in images are as oscillatory as noise is;conversely,white noise has low frequencies and therefore smooth components.Thus a separation method based on smoothness arguments only is hazardous.
1.3.The“method noise”.All denoising methods depend on a?ltering pa-rameter h.This parameter measures the degree of?ltering applied to the image.For most methods,the parameter h depends on an estimation of the noise varianceσ
2. One can de?ne the result of a denoising method D h as a decomposition of any image v as
(1.1)
v=D h v+n(D h,v),
where
1.D h v is more smooth than v
2.n(D h,v)is the noise guessed by the method.
Now,it is not enough to smooth v to ensure that n(D h,v)will look like a noise. The more recent methods are actually not contented with a smoothing,but try to recover lost information in n(D h,v)[19],[25].So the focus is on n(D h,v).
Definition1.1(Method noise).Let u be a(not necessarily noisy)image and D h a denoising operator depending on h.Then we de?ne the method noise of u as the image di?erence
(1.2)
n(D h,u)=u?D h(u).
This method noise should be as similar to a white noise as possible.In addition, since we would like the original image u not to be altered by denoising methods,the method noise should be as small as possible for the functions with the right regularity.
According to the preceding discussion,four criteria can and will be taken into account in the comparison of denoising methods:
?a display of typical artifacts in denoised images.
4 A.BUADES,B.COLL AND J.M MOREL
?a formal computation of the method noise on smooth images,evaluating how
small it is in accordance with image local smoothness.
?a comparative display of the method noise of each method on real images
withσ=2.5.We mentioned that a noise standard deviation smaller than3is
subliminal and it is expected that most digitization methods allow themselves
this kind of noise.
?a classical comparison receipt based on noise simulation:it consists of taking
a good quality image,add gaussian white noise with knownσand then com-
pute the best image recovered from the noisy one by each method.A table
of L2distances from the restored to the original can be established.The L2
distance does not provide a good quality assessment.However,it re?ects well
the relative performances of algorithms.
On top of this,in two cases,a proof of asymptotic recovery of the image can be obtained by statistical arguments.
1.4.Which methods to compare?.We had to make a selection of the de-noising methods we wished to compare.Here a di?culty arises,as most original methods have caused an abundant literature proposing many improvements.So we tried to get the best available version,but keeping the simple and genuine character of the original method:no hybrid method.So we shall analyze:
1.the Gaussian smoothing model(Gabor[16]),where the smoothness of u is measured by the Dirichlet integral |Du|2;
2.the anisotropic?ltering model(Perona-Malik[28],Alvarez et al.[1]);
3.the Rudin-Osher-Fatemi[31]total variation model and two recently proposed
iterated total variation re?nements[36,25];
4.the Yaroslavsky([42],[40])neighborhood?lters and an elegant variant,the
SUSAN?lter(Smith and Brady)[34];
5.the Wiener local empirical?lter as implemented by Yaroslavsky[40];
6.the translation invariant wavelet thresholding[8],a simple and performing
variant of the wavelet thresholding[10];
7.DUDE,the discrete universal denoiser[24]and the UINTA,Unsupervised
Information-Theoretic,Adaptive Filtering[3],two very recent new approaches;
8.the non local means(NL-means)algorithm,which we introduce here.
This last algorithm is given by a simple closed formula.Let u be de?ned in a bounded domain??R2,then
NL(u)(x)=
1
C(x) e?(G a?|u(x+.)?u(y+.)|2)(0)
h2u(y)d y,
where x∈?,G a is a Gaussian kernel of standard deviation a,h acts as a?ltering parameter and C(x)= e?(G a?|u(x+.)?u(z+.)|2)(0)
h2d z is the normalizing factor.In order to make clear the previous de?nition,we recall that
(G a?|u(x+.)?u(y+.)|2)(0)= R2G a(t)|u(x+t)?u(y+t)|2d t.
This amounts to say that NL(u)(x),the denoised value at x,is a mean of the values of all pixels whose gaussian neighborhood looks like the neighborhood of x.
1.5.What is left.We do not draw into comparison the hybrid methods,in particular the total variation+wavelets([7],[11],[17]).Such methods are signi?cant improvements of the simple methods but are impossible to draw into a benchmark:
A REVIEW OF IMAGE DENOISING ALGORIHTMS WITH A NEW ONE5 their e?ciency depends a lot upon the choice of wavelet dictionaries and the kind of
image.
Second,we do not draw into the comparison the method introduced recently by
Y.Meyer[22],whose aim it is to decompose the image into a BV part and a texture
part(the so called u+v methods),and even into three terms,namely u+v+w where
u is the BV part,v is the“texture”part belonging to the dual space of BV,denoted
by G,and w belongs to the Besov space˙B∞?1,∞,a space characterized by the fact that the wavelet coe?cients have a uniform bound.G is proposed by Y.Meyer as the right
space to model oscillatory patterns such as textures.The main focus of this method
is not denoising,yet.Because of the di?erent and more ambitious scopes of the
Meyer method,[2,37,26],which makes it parameter and implementation-dependent,
we could not draw it into the https://www.360docs.net/doc/bb7155265.html,st but not least,let us mention the
bandlets[27]and curvelets[35]transforms for image analysis.These methods also
are separation methods between the geometric part and the oscillatory part of the
image and intend to?nd an accurate and compressed version of the geometric part.
Incidentally,they may be considered as denoising methods in geometric images,as the
oscillatory part then contains part of the noise.Those methods are closely related to
the total variation method and to the wavelet thresholding and we shall be contented
with those simpler representatives.
1.6.Plan of the paper.Section2computes formally the method noise for the
best elementary local smoothing methods,namely gaussian smoothing,anisotropic
smoothing(mean curvature motion),total variation minimization and the neighbor-
hood?lters.For all of them we prove or recall the asymptotic expansion of the?lter
at smooth points of the image and therefore obtain a formal expression of the method
noise.This expression permits to characterize places where the?lter performs well
and where it fails.In section3,we treat the Wiener-like methods,which proceed by
a soft or hard threshold on frequency or space-frequency coe?cients.We examine
in turn the Wiener-Fourier?lter,the Yaroslavsky local adaptive DCT based?lters
and the wavelet threshold method.Of course the gaussian smoothing belongs to both
classes of?lters.We also describe the universal denoiser DUDE,but we cannot draw
it into the comparison as its direct application to grey level images is unpractical
so far(we discuss its feasibility).Finally,we examine the UINTA algorithms whose
principles stand close to the NL-means algorithm.In section5,we introduce the Non
Local means(NL-means)?lter.This method is not easily classi?ed in the preced-
ing terminology,since it can work adaptively in a local or non local way.We?rst
give a proof that this algorithm is asymptotically consistent(it gives back the con-
ditional expectation of each pixel value given an observed neighborhood)under the
assumption that the image is a fairly general stationary random process.The works
of Efros and Leung[13]and Levina[15]have shown that this assumption is sound
for images having enough samples in each texture patch.In section6,we compare
all algorithms from several points of view,do a performance classi?cation and ex-
plain why the NL-means algorithm shares the consistency properties of most of the
aforementioned algorithms.
2.Local smoothing?lters.The original image u is de?ned in a bounded
domain??R2,and denoted by u(x)for x=(x,y)∈R2.This continuous image is
usually interpreted as the Shannon interpolation of a discrete grid of samples,[32]and
is therefore analytic.The distance between two consecutive samples will be denoted
byε.
The noise itself is a discrete phenomenon on the sampling grid.According to
6 A.BUADES,B.COLL AND J.M MOREL
the usual screen and printing visualization practice,we do not interpolate the noise samples n i as a band limited function,but rather as a piecewise constant function,constant on each pixel i and equal to n i .We write |x |=(x 2+y 2)12and x 1.x 2=x 1x 2+y 1y 2their scalar product and
denote the derivatives of u by u x =?u ?x ,u y =?u ?y ,u xy =?2u ?x?y .The gradient of u
is written as Du =(u x ,u y )and the Laplacian of u as ?u =u xx +u yy .
2.1.Gaussian smoothing.By Riesz theorem,image isotropic linear ?ltering boils down to a convolution of the image by a linear radial kernel.The smoothing requirement is usually expressed by the positivity of the kernel.The paradigm of such
kernels is of course the gaussian x →G h (x )=1(4πh 2)e ?|x |24h 2.In that case,G h has
standard deviation h and it is easily seen that
Theorem 2.1(Gabor 1960).The image method noise of the convolution with a gaussian kernel G h is
u ?G h ?u =?h 2?u +o (h 2).
A similar result is actually valid for any positive radial kernel with bounded variance,so one can keep the gaussian example without loss of generality.The preceding estimate is valid if h is small enough.On the other hand,the noise reduction properties depend upon the fact that the neighborhood involved in the smoothing is large enough,so that the noise gets reduced by averaging.So in the following we assume that h =kε,where k stands for the number of samples of the function u and noise n in an interval of length h .The spatial ratio k must be much larger than 1to ensure a noise reduction.
The e?ect of a gaussian smoothing on the noise can be evaluated at a reference pixel i =0.At this pixel,
G h ?n (0)= i ∈I P i G h (x )n (x )d x = i ∈I
ε2G h (i )n i ,where we recall that n (x )is being interpolated as a piecewise constant function,the P i square pixels centered in i have size ε2and G h (i )denotes the mean value of the function G h on the pixel i .
Denoting by V ar (X )the variance of a random variable X ,the additivity of variances of independent centered random variables yields
V ar (G h ?n (0))= i
ε4G h (i )2σ2?σ2ε2 G h (x )2d x =ε2σ28πh 2.So we have proved
Theorem 2.2.Let n (x )be a piecewise constant white noise,with n (x )=n i on each square pixel i .Assume that the n i are i.i.d.with zero mean and variance σ2.Then the “noise residue”after a gaussian convolution of n by G h satis?es
V ar (G h ?n (0))?ε2σ2
8πh 2
.In other terms,the standard deviation of the noise,which can be interpreted as the noise amplitude,is multiplied by εh √8π.Theorems 2.1and 2.2traduce the delicate equilibrium between noise reduction and image destruction by any linear smoothing.Denoising does not alter the image
A REVIEW OF IMAGE DENOISING ALGORIHTMS WITH A NEW ONE7 at points where it is smooth at a scale h much larger than the sampling scaleε. The?rst theorem tells us that the method noise of the gaussian denoising method is zero in harmonic parts of the image.A Gaussian convolution is optimal on harmonic functions,and performs instead poorly on singular parts of u,namely edges or texture, where the Laplacian of the image is large.See Figure2.2.
2.2.Anisotropic?lters and curvature motion.The anisotropic?lter(A F) attempts to avoid the blurring e?ect of the gaussian by convolving the image u at x only in the direction orthogonal to Du(x).The idea of such?lter goes back to Perona and Malik[28]and actually again to Gabor[16].Set
A F h u(x)= G h(t)u(x+t Du(x)⊥|Du(x)|)dt,
for x such that Du(x)=0and where(x,y)⊥=(?y,x)and G h(t)=1√
2πh e?t22h2is
the one-dimensional Gauss function with variance h2.At points where Du(x)=0an isotropic gaussian mean is usually applied and the result of Theorem2.1holds at those points.If one assumes that the original image u is twice continuously di?erentiable (C2)at x,it is easily shown by a second order Taylor expansion that Theorem2.3.The image method noise of an anisotropic?lter A F h is
u(x)?A F h u(x)??1
2
h2D2u(
Du⊥
|Du|,
Du⊥
|Du|)=?
1
2
h2|Du|curv(u)(x),
where the relation holds when Du(x)=0.
By curv(u)(x),we denote the curvature,i.e.the signed inverse of the radius of curvature of the level line passing by x.When Du(x)=0,this means
curv(u)=u xx u2y?2u xy u x u y+u yy u2x
(u2x+u2y)32
.
This method noise is zero wherever u behaves locally like a one variable function, u(x,y)=f(ax+by+c).In such a case,the level line of u is locally the straight line with equation ax+by+c=0and the gradient of f may instead be very large. In other terms,with anisotropic?ltering,an edge can be maintained.On the other hand,we have to evaluate the gaussian noise reduction.This is easily done by a one-dimensional adaptation of Theorem2.2.Notice that the noise on a grid is not isotropic;so the gaussian average when Du is parallel to one coordinate axis is made roughly on
√2more samples than the gaussian average in the diagonal direction.
Theorem2.4.By anisotropic gaussian smoothing,whenεis small enough with respect to h,the noise residue satis?es
Var(A F h(n))≤
ε
√2πhσ2.
In other terms,the standard deviation of the noise n is multiplied by a factor at most equal to(ε
√2πh)1/2,this maximal value being attained in the diagonals.
Proof.Let L be the line x+t Du⊥(x)
|Du(x)|passing by x,parameterized by t∈R and denote by P i,i∈I the pixels which meet L,n(i)the noise value,constant on pixel
8 A.BUADES,B.COLL AND J.M MOREL
P i,andεi the length of the intersection of L∩P i.Denote by g(i)the average of G h(x+t Du⊥(x)
|Du(x)|)on L∩P i.Then,one has
A F h n(x)? iεi n(i)g(i).
The n(i)are i.i.d.with standard variationσand therefore
V ar(A F h(n))= iε2iσ2g(i)2≤σ2max(εi) iεi g(i)2.
This yields
Var(A F h(n))≤
√2εσ2 G h(t)2dt=ε√2πhσ2.
There are many versions of A F h,all yielding an asymptotic estimate equivalent to the one in Theorem2.3:the famous median?lter[14],an inf-sup?lter on segments centered at x[5],and the clever numerical implementation of the mean curvature equation in[21].So all of those?lters have in common the good preservation of edges, but they perform poorly on?at regions and are worse there than a gaussian blur.This fact derives from the comparison of the noise reduction estimates of Theorems2.1and 2.4,and is experimentally patent in Figure2.2.
2.3.Total variation.The Total variation minimization was introduced by Rudin, Osher and Fatemi[30,31].The original image u is supposed to have a simple ge-ometric description,namely a set of connected sets,the objects,along with their smooth contours,or edges.The image is smooth inside the objects but with jumps across the boundaries.The functional space modelling these properties is BV(?),the space of integrable functions with?nite total variation T V?(u)= |Du|,where Du is assumed to be a Radon measure.Given a noisy image v(x),the above mentioned authors proposed to recover the original image u(x)as the solution of the constrained minimization problem
arg min
u T V?(u),
(2.1)
subject to the noise constraints
?(u(x)?v(x))d x=0and ?|u(x)?v(x)|2d x=σ2.
The solution u must be as regular as possible in the sense of the total variation, while the di?erence v(x)?u(x)is treated as an error,with a prescribed energy.The constraints prescribe the right mean and variance to u?v,but do not ensure that it be similar to a noise(see a thorough discussion in[22]).The preceding problem is naturally linked to the unconstrained problem
arg min
u T V?(u)+λ ?|v(x)?u(x)|2d x,
(2.2)
A REVIEW OF IMAGE DENOISING ALGORIHTMS WITH A NEW ONE9 for a given Lagrange multiplierλ.The above functional is strictly convex and lower semicontinuous with respect to the weak-star topology of BV.Therefore the minimum exists,is unique and computable(see(e.g.)[6].)The parameterλcontrols the trade o?between the regularity and?delity terms.Asλgets smaller the weight of the regularity term increases.Thereforeλis related to the degree of?ltering of the solution of the minimization problem.Let us denote by TVFλ(v)the solution of problem(2.2)for a given value ofλ.The Euler Lagrange equation associated with the minimization problem is given by
(u(x)?v(x))?
1
2λ
curv(u)(x)=0,
(see[30]).Thus,
Theorem2.5.The image method noise of the total variation minimization(2.2) is
u(x)?TVFλ(u)(x)=?
1
2λ
curv(TVFλ(u))(x).
As in the anisotropic case,straight edges are maintained because of their small curvature.However,details and texture can be over smoothed ifλis too small,as is shown in Figure2.2.
2.4.Iterated Total Variation re?nement.In the original TV model the removed noise,v(x)?u(x),is treated as an error and is no longer studied.In practice, some structures and texture are present in this error.Several recent works have tried to avoid this e?ect[36,25].
2.4.1.The Tadmor et al.approach.In[36],the authors have proposed to use the Rudin-Osher-Fatemi iteratively.They decompose the noisy image,v=u0+n0 by the total variation model.So taking u0to contain only geometric information,they decompose by the very same model n0=u1+n1,where u1is assumed to be again a geometric part and n1contains less geometric information than n0.Iterating this process,one obtains u=u0+u1+u2+...+u k as a re?ned geometric part and n k as the noise residue.This strategy is in some sense close to the matching pursuit methods [20].Of course,the weight parameter in the Rudin-Osher-Fatemi has to grow at each iteration and the authors propose a geometric seriesλ,2λ,....,2kλ.In that way,the extraction of the geometric part n k becomes twice more asking at each step.Then, the new algorithm is as follows:
1.Starting with an initial scaleλ=λ0,
v=u0+n0,[u0,n0]=arg min
v=u+n |Du|+λ0 |v(x)?u(x)|2d x.
2.Proceed with successive applications of the dyadic re?nement n j=u j+1+
n j+1,
[u j+1,n j+1]=arg min
n j=u+n |Du|+λ02j+1 |n j(x)?u(x)|2d x.
3.After k steps,we get the following hierarchical decomposition of v
v=u0+n0
=u0+u1+n1
=.....
=u0+u1+...+u k+n k.
10 A.BUADES,B.COLL AND J.M MOREL
The denoised image is given by the partial sum k j =0u j and n k is the noise residue.
This is a multilayered decomposition of v which lies in an intermediate scale of spaces,in between BV and L 2.Some theoretical results on the convergence of this expansion are presented in [36].
2.4.2.The Osher et al.approach.The second algorithm due to Osher et al.[25],also consists of an iteration of the original model.The new algorithm is as follows.
1.First solve the original TV model
u 1=arg min u ∈BV
|?u (x )|d x +λ (v (x )?u (x ))2d x ,to obtain the decomposition v =u 1+n 1.
2.Perform a correction step to obtain
u 2=arg min u ∈BV
|?u (x )|d x +λ (v (x )+n 1(x )?u (x ))2d x ,where n 1is the noise estimated by the ?rst step.The correction step adds this ?rst estimate of the noise to the original image and raises the following decomposition v +n 1=u 2+n 2.
3.Iterate :compute u k +1as a minimizer of the modi?ed total variation mini-mization,
u k +1=arg min u ∈BV
|?u (x )|d x +λ (v (x )+n k (x )?u (x ))2d x ,where
v +n k =u k +1+n k +1.
Some results are presented in [25]which clarify the nature of the above sequence:?{u k }k converges monotonically in L 2to v ,the noisy image,as k →∞.
?{u k }k approaches the noisy free image monotonically in the Bregman distance associated with the BV seminorm,at least until u ˉk ?u ≤σ2,where u is the original image and σis the standard deviation of the added noise.
These two results indicate how to stop the sequence and choose u ˉk .It is enough to proceed iteratively until the result gets noisier or the distance u ˉk ?u 2gets smaller than σ2.The new solution has more details preserved,as Figure 2.2shows.
The above iterated denoising strategy being quite general,one can make the computations for a linear denoising operator T as well.In that case,this strategy
T (v +n 1)=T (v )+T (n 1)
amounts to say that the ?rst estimated noise n 1is ?ltered again and its smooth components added back to the original,which is in fact the Tadmor et al.strategy.
2.5.Neighborhood ?lters.The previous ?lters are based on a notion of spa-tial neighborhood or proximity.Neighborhood ?lters instead take into account grey level values to de?ne neighboring pixels.In the simplest and more extreme case,the denoised value at pixel i is an average of values at pixels which have a grey level value close to u (i ).The grey level neighborhood is therefore
B (i,h )={j ∈I |u (i )?h
A REVIEW OF IMAGE DENOISING ALGORIHTMS WITH A NEW ONE 11
This is a fully non-local algorithm,since pixels belonging to the whole image are used for the estimation at pixel i .This algorithm can be written in a more continuous form
NF h u (x )=1C (x ) ?
u (y )e ?|u (y )?u (x )|2h 2d y ,where ??R 2is an open and bounded set,and C (x )= ?e ?|u (y )?u (x )|2
h 2d y is the
normalization factor.The ?rst question to address here is the consistency of such a ?lter,namely how close the denoised version is to the original when u is smooth.
Lemma 2.6.Suppose u is Lipschitz in ?and h >0,then C (x )≥O (h 2).
Proof .Given x ,y ∈?,by the Mean Value Theorem,|u (x )?u (y )|≤K |x ?y |for
some real constant K .Then,C (x )= ?e ?|u (y )?u (x )|2h 2d y ≥ B (x ,h )e ?|u (y )?u (x )|2h 2d y ≥e ?K 2O (h 2).
Proposition 2.7.(Method noise estimate).Suppose u is a Lipschitz bounded function on ?,where ?is an open and bounded domain of R 2.Then |u (x )?NF h u (x )|=O (h √?log h ),for h small,0 D c h e ?|u (y )?u (x )|2h 2 |u (y )?u (x )|d y .By one hand,considering that D h e ?|u (y )?u (x )|2h 2d y ≤C (x )and |u (y )?u (x )|≤Bh for y ∈D h one one sees that the ?rst term is bounded by Bh .On the other hand,considering that e ?|u (y )?u (x )|2h 2≤e ?B 2for y /∈D h , D c h |u (y )?u (x )|d y is bounded and by Lemma 2.6,C ≥O (h 2),one deduces that the second term has an order O (h ?2e ?B 2).Finally,choosing B such that B 2=?3log h yields |u (x )?NF h u (x )|≤Bh +O (h ?2e ?B 2)=O (h ?log h )+O (h )and so the method noise has order O (h √?log h ).The Yaroslavsky ([40,42])neighborhood ?lters consider mixed neighborhoods B (i,h )∩B ρ(i ),where B ρ(i )is a ball of center i and radius ρ.So the method takes an average of the values of pixels which are both close in grey level and spatial distance.This ?lter can be easily written in a continuous form as, YNF h,ρ(x )=1C (x ) B ρ(x ) u (y )e ?|u (y )?u (x )|2h 2d y ,where C (x )= B ρ(x )e ?|u (y )?u (x )|2 h 2d y is the normalization factor.In [34]the authors present a similar approach where they do not consider a ball of radius ρbut they weigh the distance to the central pixel,obtaining the following close formula, 1C (x ) u (y )e ?|y ?x |2ρ2e ?|u (y )?u (x )|2h 2d y , 12 A.BUADES,B.COLL AND J.M MOREL where C(x)= e?|y?x|2ρ2e?|u(y)?u(x)|2h2d y is the normalization factor. First,we study the method noise of the YNF h,ρin the1D case.In that case,u denotes a one dimensional signal. Theorem2.8.Suppose u∈C2((a,b)),a,b∈R.Then,for0<ρ< u(s)?YNF h,ρu(s)??ρ2 2 f( ρ h|u′(s)|)u′′(s), where f(t)=1 3?35t2 1?1 3 t2 . Proof.Let s∈(a,b)and h,ρ∈R+.Then u(s)?YNF h,ρ(s)=? 1 ρ?ρe?(u(s+t)?u(s))2h2dt ρ?ρ(u(s+t)?u(s))e?(u(s+t)?u(s))2h2dt. If we take the Taylor expansion of u(s+t)and the exponential function e?x2and we integrate,then we obtain that u(s)?YNF h,ρ(s)??ρ3u′′ 3?3ρ 5u′2u′′ 5h2 2h?2ρ3u′2 3h2 , forρsmall enough.The method noise follows from the above expression. The previous result shows that the neighborhood?ltering method noise is pro- portional to the second derivative of the signal.That is,it behaves like a weighted heat equation.The function f gives the sign and the magnitude of this heat equation. Where the function f takes positive values,the method noise behaves as a pure heat equation,while where it takes negative values,the method noise behaves as a reverse heat equation.The zeros and the discontinuity points of f represent the singular points where the behavior of the method changes.The magnitude of this change is much larger near the discontinuities of f producing an ampli?ed shock e?ect.Figure 2.1displays one experiment with the one dimensional neighborhood?lter.We iterate the algorithm on a sine signal and illustrate the shock e?ect.For the two intermediate iterations u n+1,we display the signal f(ρ h|u′n|)which gives the sign and magnitude of the heat equation at each point.We can see that the positions of the discontinuities of f(ρ h|u′n|)describe exactly the positions of the shocks in the further iterations and the ?nal state.These two examples corroborate Theorem2.8and show how the function f totally characterizes the performance of the one dimensional neighborhood?lter. Next we give the analogous result for2D images. Theorem2.9.Suppose u∈C2(?),??R2.Then,for0<ρ< u(x)?YNF h,ρ(x)??ρ2 8 (g( ρ h|Du|)uηη+h( ρ h|Du|)uξξ), where uηη=D2u(Du |Du|, Du |Du|),uξξ=D2u( Du⊥ |Du|, Du⊥ |Du|) A REVIEW OF IMAGE DENOISING ALGORIHTMS WITH A NEW ONE 13 (a) (b) (c) (d) (e)(f) Fig.2.1.One dimensional neighborhood ?ltering experience.We iterate the ?lter on the sine signal until it converges to a steady state.We show the input signal (a)and the ?nal state (b).The ?gures (e)and (f)display two intermediate states.Figures (c)and (d)display the signal f (ρh |u ′n |)which gives the magnitude and signal of the heat equation leading to ?gures (e)and (f).These two signals describe exactly the positions of the shocks in the further iterations and the ?nal state.and g (t )=1?32t 21?14t 2,h (t )=1?12t 21?14 t 2.Proof .Let x ∈?and h,ρ∈R +.Then u (x )?YNF h,ρ(x )=?1 B ρ(0)e ?(u (x +t )?u (x ))2h 2d t B ρ(0) (u (x +t )?u (x ))e ?(u (x +t )?u (t ))2h 2d t .We take the Taylor expansion of u (x +t ),and the exponential function e ?y 2.Then, we take polar coordinates and integrate,obtaining u (x )?YNF h,ρ(x )?1πρ2?ρ4π4h 2(u 2x +u 2y ) πρ48?u ?πρ616h 2 u 2x u xx +u 2y u xx ++u 2x u yy +u 2x u xx ?πρ68h 2(u 2x u xx +2u x u y u xy +u 2y u yy ) 14 A.BUADES,B.COLL AND J.M MOREL for ρsmall enough.By grouping the terms of above expression,we get the desired result. The neighborhood ?ltering method noise can be written as the sum of a di?usion term in the tangent direction u ξξ,plus a di?usion term in the normal direction,u ηη.The sign and the magnitude of both di?usions depend on the sign and the magnitude of the functions g and h .Both functions can take positive and negative values.There-fore,both di?usions can appear as a directional heat equation or directional reverse heat equation depending on the value of the gradient.As in the one dimensional case,the algorithm performs like a ?ltering /enhancing algorithm depending on the value of the gradient.If B 1=√2/√3and B 2=√2respectively denote the zeros of the functions g and h ,we can distinguish the following cases: ?When 0<|Du | [28].In a ?rst step,a heat equation is applied but when |Du |>B 1h ρthe normal di?usion turns into a reverse di?usion enhancing the edges,while the tangent di?usion stays positive. ?When |Du |>B 2h ρthe algorithm di?ers from the Perona-Malik ?lter.A heat equation or a reverse heat equation is applied depending on the value of the gradient.The change of behavior between these two dynamics is marked by an asymptotical discontinuity leading to an ampli?ed shock e?ect. Fig.2.2.Denoising experience on a natural image.From left to right and from top to bot-tom:noisy image (standard deviation 20),gaussian convolution,anisotropic ?lter,total variation minimization,Tadmor et al.iterated total variation,Osher et al.iterated total variation and the Yaroslavsky neighborhood ?lter. 3.Frequency domain ?lters.Let u be the original image de?ned on the grid I .The image is supposed to be modi?ed by the addition of a signal independent white noise N .N is a random process where N (i )are i.i.d,zero mean and have constant variance σ2.The resulting noisy process depends on the random noise component,and therefore is modelled as a random ?eld V , V (i )=u (i )+N (i ). (3.1)Given a noise observation n (i ),v (i )denotes the observed noisy image, v (i )=u (i )+n (i ). (3.2) A REVIEW OF IMAGE DENOISING ALGORIHTMS WITH A NEW ONE15 Let B={gα}α∈A be an orthogonal basis of R|I|.The noisy process is transformed as (3.3) V B(α)=u B(α)+N B(α), where V B(α)= V,gα ,u B(α)= u,gα ,N B(α)= N,gα are the scalar products of V,u and N with gα∈B.The noise coe?cients N B(α) remain uncorrelated and zero mean,but the variances are multiplied by gα 2, E[N B(α)N B(β)]= m,n∈I gα(m)gβ(n)E[N(m)N(n)] = gα,gβ σ2=σ2 gα 2δ[α?β]. Frequency domain?lters are applied independently to every transform coe?cient V B(α)and then the solution is estimated by the inverse transform of the new coef-?cients.Noisy coe?cients V B(α)are modi?ed to a(α)V B(α).This is a non linear algorithm because a(α)depends on the value V B(α).The inverse transform yields the estimate ?U=DV= α∈A a(α)V B(α)gα. (3.4) D is also called a diagonal operator.Let us look for the frequency domain?lter D which minimizes a certain estimation error.This error is based on the square euclidean distance,and it is averaged over the noise distribution. Definition3.1.Let u be the original image,N a white noise and V=u+N. Let D be a frequency domain?lter.De?ne the risk of D as (3.5) r(D,u)=E{||u?DV||2}, where the expectation is taken over the noise distribution. The following theorem,which is easily proved,gives the diagonal operator D inf that minimizes the risk, r(D,u). D inf=arg min D Theorem3.2.The operator D inf which minimizes the risk is given by the family {a(α)}α,where (3.6) a(α)=|u B(α)|2 |u B(α)|2+ gα 2σ2, and the corresponding risk is (3.7) r inf(u)= s∈S gα 4|u B(α)|2σ2 |u B(α)|2+ gα 2σ2. 16 A.BUADES,B.COLL AND J.M MOREL The previous optimal operator attenuates all noisy coe?cients in order to mini-mize the risk.If one restricts a(α)to be0or1,one gets a projection operator.In that case,a subset of coe?cients is kept and the rest gets cancelled.The projection operator that minimizes the risk r(D,u)is obtained by the following family{a(α)}α, where a(α)= 1|u B(α)|2≥||gα||2σ2 0otherwise and the corresponding risk is r p(u)= ||gα||2min(|u B(α)|2,||gα||2σ2). Note that both?lters are ideal operators because they depend on the coe?cients u B(α)of the original image,which are not known.We call,as classical,Fourier Wiener Filter the optimal operator(3.6)where B is a Fourier Basis.This is an ideal ?lter,since it uses the(unknown)Fourier transform of the original image.By the use of the Fourier basis(see Figure3.1),global image characteristics may prevail over local ones and create spurious periodic patterns.To avoid this e?ect,the basis must take into account more local features,as the wavelet and local DCT transforms do. The search for the ideal basis associated with each image still is open.At the moment, the way seems to be a dictionary of basis instead of one single basis,[19]. Fig.3.1.Fourier Wiener?lter experiment.Top Left:Degraded image by an additive white noise ofσ=15.Top Right:Fourier Wiener?lter solution.Down:Zoom on three di?erent zones of the solution.The image is?ltered as a whole and therefore a uniform texture is spread all over the image. 3.1.Local adaptive?lters in transform Domain.The local adaptive?lters have been introduced by L.Yaroslavsky[42,41].In this case,the noisy image is analyzed in a moving window and in each position of the window its spectrum is computed and modi?ed.Finally,an inverse transform is used to estimate only the signal value in the central pixel of the window. A REVIEW OF IMAGE DENOISING ALGORIHTMS WITH A NEW ONE17 Let i∈I be a pixel and W=W(i)a window centered in i.Then the DCT transform of W is computed and modi?ed.The original image coe?cients of W, u B,W(α)are estimated and the optimal attenuation of Theorem3.2is applied.Finally, only the center pixel of the restored window is used.This method is called the Empirical Wiener Filter.In order to approximate u B,W(α)one can take averages on the additive noise model,that is, E|V B,W(α)|2=|u B,W(α)|2+σ2||gα||2. Denoting byμ=σ||gα||,the unknown original coe?cients can be written as |u B,W(α)|2=E|V B,W(α)|2?μ2. The observed coe?cients|v B,W(α)|2are used to approximate E|V B,W(α)|2,and the estimated original coe?cients are replaced in the optimal attenuation,leading to the family{a(α)}α,where . a(α)=max 0,|v B,W(α)|2?μ2 |v B,W(α)|2 Denote by EW Fμ(i)the?lter given by the previous family of coe?cients.The method noise of the EW Fμ(i)is easily computed,as proved in the following theorem. Theorem3.3.Let u be an image de?ned in a grid I and let i∈I be a pixel. Let W=W(i)be a window centered in the pixel i.Then the method noise of the EWFμ(i)is given by u(i)?EWFμ(i)= α∈Λv B,W(α)gα(i)+ α/∈Λμ2|v B,W(α)|2v B,W(α)gα(i). whereΛ={α||v B,W(α)|<μ}. The presence of an edge in the window W will produce a great amount of large coe?cients and as a consequence,the cancelation of these coe?cients will produce oscillations.Then,spurious cosines will also appear in the image under the form of chessboard patterns,see Figure3.2. 3.2.Wavelet thresholding.Let B={gα}α∈A be an orthonormal basis of wavelets[20].Let us discuss two procedures modifying the noisy coe?cients,called wavelet thresholding methods(D.Donoho et al.[10]).The?rst procedure is a projec-tion operator which approximates the ideal projection(3.6).It is called hard thresh-olding,and cancels coe?cients smaller than a certain thresholdμ, a(α)= 1|v B(α)|>μ 0otherwise Let us denote this operator by HWTμ(v).This procedure is based on the idea that the image is represented with large wavelet coe?cients,which are kept,whereas the noise is distributed across small coe?cients,which are canceled.The performance of the method depends on the capacity of approximating u by a small set of large coe?cients.Wavelets are for example an adapted representation for smooth functions. Theorem3.4.Let u be an image de?ned in a grid I.The method noise of a hard thresholding HWTμ(u)is u?HWTμ(u)= {α||u B(α)|<μ}u B(α)gα 18 A.BUADES,B.COLL AND J.M MOREL Unfortunately,edges lead to a great amount of wavelet coe?cients lower than the threshold,but not zero.The cancelation of these wavelet coe?cients causes small oscillations near the edges,i.e.a Gibbs-like phenomenon.Spurious wavelets can also be seen in the restored image due to the cancelation of small coe?cients :see Figure 3.2.This artifact will be called:wavelet outliers ,as it is introduced in [12].D.Donoho [9]showed that these e?ects can be partially avoided with the use of a soft thresholding, a (α)= v B (α)?sgn (v B (α))μv B (α)|v B (α)|≥μ0otherwise which will be denoted by SWT μ(v ).The continuity of the soft thresholding operator better preserves the structure of the wavelet coe?cients,reducing the oscillations near discontinuities.Note that a soft thresholding attenuates all coe?cients in order to reduce the noise,as an ideal operator does.As we shall see at the end of this paper,the L 2norm of the method noise is lessened when replacing the hard by a soft threshold.See Figures 3.2and 6.3for a comparison of the both method noises. Theorem 3.5.Let u be an image de?ned in a grid I .The method noise of a soft thresholding SWT μ(u )is u ?SWT μ(u )= {α||u B (α)|<μ}u B (α)g α+μ {α||u B (α)|>μ} sgn (u B (α))g α A simple example can show how to ?x the threshold μ.Suppose the original image u is zero,then v B (α)=n B (α),and therefore the threshold μmust be taken over the maximum of noise coe?cients to ensure their suppression and the recovery of the original image.It can be shown that the maximum amplitude of a white noise has a high probability of being smaller than σ 2log |I |.It can be proved that the risk of a wavelet thresholding with the threshold μ=σ 2log |I |is near the risk r p of the optimal projection,see [10,20].Theorem 3.6.The risk r t (u )of a hard or soft thresholding with the threshold μ=σ 2log |I |is such that for all |I |≥4 r t (u )≤(2log |I |+1)(σ2+r p (u )). (3.8)The factor 2log |I |is optimal among all the diagonal operators in B ,that is,lim |I |?>∞inf D ∈D B sup u ∈R |I | E {||u ?DV ||2}σ2+r p (u )12log |I |=1.(3.9)In practice the optimal threshold μis very high and cancels too many coe?cients not produced by the noise.A threshold lower than the optimal is used in the experi-ments and produces much better results,see Figure 3.2.For a hard thresholding the threshold is ?xed to 3?σ.For a soft thresholding this threshold still is too high ;it is better ?xed at 32σ. 3.3.Translation invariant wavelet thresholding.R.Coifman and D.Donoho [8]improved the wavelet thresholding methods by averaging the estimation of all trans-lations of the degraded signal.Calling v p (i )the translated signal v (i ?p ),the wavelet A REVIEW OF IMAGE DENOISING ALGORIHTMS WITH A NEW ONE 19 coe?cients of the original and translated signals can be very di?erent,and they are not related by a simple translation or permutation, v p B (α)= v (n ?p ),g α(n ) = v (n ),g α(n +p ) .The vectors g α(n +p )are not in general in the basis B ={g α}α∈A ,and therefore the estimation of the translated signal is not related to the estimation of v .This new algorithm yields an estimate ?u p for every translated v p of the original image,?u p =Dv p = α∈A a (α)v p B (α)g α. (3.10)The translation invariant thresholding is obtained by averaging all these estimators after a translation in the inverse sense,1|I | p ∈I ?u p (i +p ),(3.11) and will be denoted by TIWT (v ). The Gibbs e?ect is considerably reduced by the translation invariant wavelet thresholding,(see Figure 3.2),because the average of di?erent estimations of the image reduces the oscillations.This is therefore the version we shall use in the comparison section.Recently,S.Durand and M.Nikolova [12]have actually proposed an e?cient variational method ?nding the best compromise to avoid the three common artifacts in TV methods and wavelet thresholding,namely the stair-casing,the Gibbs e?ect and the wavelet outliers.Unfortunately,we couldn’t draw the method into the comparison. Fig.3.2.Denoising experiment on a natural image.From left to right and from top to bottom:noisy image (standard deviation 20),Fourier Wiener ?lter (ideal ?lter),the DCT empirical Wiener ?lter,the wavelet hard thresholding,the soft wavelet thresholding and the translation invariant wavelet hard thresholding. 4.Statistical neighborhood approaches.The methods we are going to con-sider are very recent attempts to take advantage of an image model learned from the image itself.More speci?cally,these denoising methods attempt to learn the statisti-cal relationship between the image values in a window around a pixel and the pixel value at the window center. 20 A.BUADES,B.COLL AND J.M MOREL 4.1.DUDE,a universal denoiser.The recent work by Ordentlich et al.[39] has led to the proposition of a“universal denoiser”for digital images.The authors assume that the noise model is fully known,namely the probability transition matrix Π(a,b),where a,b∈A,the?nite alphabet of all possible values for the image.In order to?x ideas,we shall assume as in the rest of this paper that the noise is additive gaussian,in which case one simply hasΠ(a,b)=1 √2πσe?(a?b)2 2σ2for the probability of observing b when the real value was a.The authors also?x an error costΛ(a,b) which,to?x ideas,we can take to be a quadratic functionΛ(a,b)=(a?b)2,namely the cost of mistaking a for b. The authors?x a neighborhood shape,say,a square discrete window deprived of its center i,?N i=N i\{i}around each pixel i.Then the question is:once the image has been observed in the window?N i,what is the best estimate we can make from the observation of the full image? The authors propose the following algorithm: ?Compute,for each possible value b of u(i)the number of windows N j in the image such the restrictions of u to?N j and?N i coincide and the observed value at the pixel j is b.This number is called m(b,N i)and the line vector (m(b,N i))b∈A is denoted by m(N i). ?Then,compute the denoised value of u at i as ?u(i)=arg min m(N i)Π?1(Λb?Πu(i)), b∈A where w?v=(w(b)v(b))denotes the vector obtained by multiplying each component of u by each component of v,u(i)is the observed value at i,and we denote by X a the a-column of a matrix X. The authors prove that this denoiser is universal in the sense“of asymptotically achieving,without access to any information on the statistics of the clean signal,the same performance as the best denoiser that does have access to this information”.In [24]the authors present an implementation valid for binary images with an impulse noise,with excellent results.The reason of these limitations in implementation are clear:?rst the matrixΠis of very low dimension and invertible for impulse noise.If instead we consider as above a gaussian noise,then the application ofΠ?1amounts to deconvolve a signal by a gaussian,which is a rather ill-conditioned method.All the same,it is doable,while the computation of m certainly is not for a large alphabet,like the one involved in grey tone images(256values).Even supposing that the learning window N i has the minimal possible size of9,the number of possible such windows is about2569which turns out to be much larger than the number of observable windows in an image(whose typical size amounts to106pixels).Actually,the number of samples can be made signi?cantly smaller by quantizing the grey level image and by noting that the window samples are clustered.Anyway,the direct observation of the number m(N i)in an image is almost hopeless,particularly if it is corrupted by noise. 4.2.The UINTA algorithm.Awate and Whitaker[3]have proposed a method whose principles stand close to the the NL-means algorithm,since,as in Dude,the method involves comparison between subwindows to estimate a restored value.The objective of the algorithm”UINTA,for unsupervised information theoretic adaptive ?lter”,is to denoise the image decreasing the randomness of the image.The algorithm proceeds as follows: ?Assume that the(2d+1)×(2d+1)windows in the image are realizations of a random vector Z.The probability distribution function of Z is estimated 尊重的素材(为人处世) 思路 人与人之间只有互相尊重才能友好相处 要让别人尊重自己,首先自己得尊重自己 尊重能减少人与人之间的摩擦 尊重需要理解和宽容 尊重也应坚持原则 尊重能促进社会成员之间的沟通 尊重别人的劳动成果 尊重能巩固友谊 尊重会使合作更愉快 和谐的社会需要彼此间的尊重 名言 施与人,但不要使对方有受施的感觉。帮助人,但给予对方最高的尊重。这是助人的艺术,也是仁爱的情操。—刘墉 卑己而尊人是不好的,尊己而卑人也是不好的。———徐特立 知道他自己尊严的人,他就完全不能尊重别人的尊严。———席勒 真正伟大的人是不压制人也不受人压制的。———纪伯伦 草木是靠着上天的雨露滋长的,但是它们也敢仰望穹苍。———莎士比亚 尊重别人,才能让人尊敬。———笛卡尔 谁自尊,谁就会得到尊重。———巴尔扎克 人应尊敬他自己,并应自视能配得上最高尚的东西。———黑格尔 对人不尊敬,首先就是对自己的不尊敬。———惠特曼 每当人们不尊重我们时,我们总被深深激怒。然而在内心深处,没有一个人十分尊重自己。———马克·吐温 忍辱偷生的人,绝不会受人尊重。———高乃依 敬人者,人恒敬之。———《孟子》 人必自敬,然后人敬之;人必自侮,然后人侮之。———扬雄 不知自爱反是自害。———郑善夫 仁者必敬人。———《荀子》 君子贵人而贱己,先人而后己。———《礼记》 尊严是人类灵魂中不可糟蹋的东西。———古斯曼 对一个人的尊重要达到他所希望的程度,那是困难的。———沃夫格纳 经典素材 1元和200元 (尊重劳动成果) 香港大富豪李嘉诚在下车时不慎将一元钱掉入车下,随即屈身去拾,旁边一服务生看到了,上前帮他拾起了一元钱。李嘉诚收起一元钱后,给了服务生200元酬金。 这里面其实包含了钱以外的价值观念。李嘉诚虽然巨富,但生活俭朴,从不挥霍浪费。他深知亿万资产,都是一元一元挣来的。钱币在他眼中已抽象为一种劳动,而劳动已成为他最重要的生存方式,他的所有财富,都是靠每天20小时以上的劳动堆积起来的。200元酬金,实际上是对劳动的尊重和报答,是不能用金钱衡量的。 富兰克林借书解怨 (尊重别人赢得朋友) 那一刻我感受到了幸福 本文是关于初中作文的那一刻我感受到了幸福,感谢您的阅读! 每个人民的心中都有一粒幸福的种子,当它拥有了雨水的滋润和阳光的沐浴,它就会绽放出最美丽的姿态。那一刻,我们都能够闻到幸福的芬芳,我们都能够感受到幸福的存在。 在寒假期间,我偶然在授索电视频道,发现(百家讲坛)栏目中大学教授正在解密幸福,顿然引起我的好奇心,我放下了手中的遥控器,静静地坐在电视前,注视着频道上的每一个字,甚至用笔急速记在了笔记本上。我还记得,那位大学教授讲到了一个故事:一位母亲被公司升职到外国工作,这位母亲虽然十分高兴,但却又十分无奈,因为她的儿子马上要面临中考了,她不能撇下儿子迎接中考的挑战,于是她决定拒绝这了份高薪的工作,当有人问她为什么放弃这么好的机会时,她却毫无遗憾地说,纵然我能给予儿子最贵的礼物,优异的生活环境,但我却无当给予他关键时刻的那份呵护与关爱,或许以后的一切会证明我的选择是正确的。听完这样一段故事,我心中有种说不出的感觉,刹那间,我仿拂感觉那身边正在包饺子的妈妈,屋里正在睡觉的爸爸,桌前正在看小说的妹妹给我带来了一种温馨,幸福感觉。正如教授所说的那种解密幸福。就要选择一个明确的目标,确定自已追求的是什么,或许那时我还不能完全诠释幸福。 当幸福悄悄向我走来时,我已慢慢明白,懂得珍惜了。 那一天的那一刻对我来说太重要了,原本以为出差在外的父母早已忘了我的生日,只有妹妹整日算着日子。我在耳边唠叨个不停,没想到当日我失落地回到家中时,以为心中并不在乎生日,可是眼前的一切,让我心中涌现的喜悦,脸上露出的微笑证明我是在乎的。 爸爸唱的英文生日快乐歌虽然不是很动听,但爸爸对我的那份爱我听得很清楚,妈妈为我做的长寿面,我细细的品尝,吃出了爱的味道。妹妹急忙让我许下三个愿望,嘴里不停的唠叨:我知道你的三个愿望是什么?我问:为什么呀!我们是一家人,心连心呀!她高兴的说。 那一刻我才真正解开幸福的密码,感受到了真正的幸福,以前我无法理解幸福,即使身边有够多的幸福也不懂得欣赏,不懂得珍惜,只想拥有更好更贵的,其实幸福比物质更珍贵。 那一刻的幸福就是爱的升华,许多时候能让我们感悟幸福不是名利,物质。而是在血管里涌动着的,漫过心底的爱。 也许每一个人生的那一刻,就是我们幸运的降临在一个温馨的家庭中,而不是降临在孤独的角落里。 家的感觉就是幸福的感觉,幸福一直都存在于我们的身边! 关于我的幸福作文八篇汇总 幸福在每个人的心中都不一样。在饥饿者的心中,幸福就是一碗香喷喷的米饭;在果农的心中,幸福就是望着果实慢慢成熟;在旅行者的心中,幸福就是游遍世界上的好山好水。而在我的心中,幸福就是每天快快乐乐,无忧无虑;幸福就是朋友之间互相帮助,互相关心;幸福就是在我生病时,母亲彻夜细心的照顾我。 幸福在世间上的每个角落都可以发现,只是需要你用心去感受而已。 记得有一次,我早上出门走得太匆忙了,忘记带昨天晚上准备好的钢笔。老师说了:“今天有写字课,必须要用钢笔写字,不能用水笔。”我只好到学校向同学借了。当我来到学校向我同桌借时,他却说:“我已经借别人了,你向别人借吧!”我又向后面的同学借,可他们总是找各种借口说:“我只带了一枝。”问了三四个人,都没有借到,而且还碰了一鼻子灰。正当我急的像热锅上的蚂蚁团团转时,她递给了我一枝钢笔,微笑的对我说:“拿去用吧!”我顿时感到自己是多么幸福!在我最困难的时候,当别人都不愿意帮助我的时候,她向我伸出了援手。 幸福也是无时无刻都在身旁。 当我生病的时候,高烧持续不退时,是妈妈在旁边细心 的照顾我,喂我吃药,甚至一夜寸步不离的守在我的床边,直到我苏醒。当我看见妈妈的眼睛布满血丝时,我的眼眶在不知不觉地湿润了。这时我便明白我有一个最疼爱我的妈妈,我是幸福的! 幸福就是如此简单!不过,我们还是要珍惜眼前的幸福,还要给别人带来幸福,留心观察幸福。不要等幸福悄悄溜走了才发现,那就真的是后悔莫及了! 这就是我拥有的幸福,你呢? 悠扬的琴声从房间里飘出来,原来这是我在弹钢琴。优美的旋律加上我很强的音乐表现力让一旁姥爷听得如醉如痴。姥爷说我是幸福的,读了《建设幸福中国》我更加体会到了这一点。 儿时的姥爷很喜欢读书,但当时家里穷,据姥爷讲那时上学可不像现在。有点三天打鱼两天晒网,等地里农活忙了太姥爷就说:“别去念书了,干地里的活吧。”干活时都是牛马拉车,也没机器,效率特别低。还要给牲口拔草,喂草,拾柴火,看书都是抽空看。等农闲时才能背书包去学校,衣服更是老大穿了,打补丁老二再接着穿,只有盼到过年时才有能换上件粗布的新衣服。写字都是用石板,用一次擦一次,那时还没有电灯,爱学习的姥爷在昏暗的煤油灯下经常被灯火不是烧了眉毛就是燎了头发。没有电灯更没有电视,没有电视更没有见过钢琴,只知道钢琴是贵族家用的。 小学生作文《感悟幸福》范文五篇 小草说,幸福就是大地增添一份绿意;阳光说,幸福就是撒向人间的温暖;甘露说,幸福就是滋润每一个生命。下面是为大家带来的有关幸福650字优秀范文,希望大家喜欢。 感悟幸福650字1 生活就像一部壮丽的交响曲,它是由一篇一篇的乐章组成的,有喜、有怒、有哀、有乐。每一个人都有自己丰富多彩的生活,我也有自己的生活。我原本以为,吃可口的牛排,打电脑游戏,和朋友开心玩乐就是幸福。可是,我错了,幸福并不仅仅如此。 记得有一次,我放学回到家里放下书包就拿起一包饼干来吃。吃着吃着,突然我觉得牙齿痛了起来,而且越来越痛,痛得我连饼干也咬不动了。我放下饼干,连忙去拿了一面镜子来看。原来这又是那一颗虫牙在“作怪”。“哎哟哟,哎哟哟,痛死我了……”我不停地说着。渐渐地,那牙疼得越来越厉害,疼得我坐立不安,直打滚。后来在妈妈的陪伴下去了医院,治好了那颗虫牙。跨出医院大门时,我觉得心情出奇的好,天空格外的蓝,路边的樟树特别的绿,看什么都顺眼,才猛然一悟,幸福是简单而平凡的,身体健康就是一种幸福! 这学期我发现我的英语退步了,我决定要把这门功课学好,于是,我每天回 家做完作业后,都抽出半小时时间复习英语,在课上也听得特别认真,一遇到不懂的题目主动请教老师。经过一段时间的努力,终于,在上次考试的时候,我考了97分。妈妈表扬了我,我心里美滋滋的。我明白了经过自己的努力享受到成功的喜悦,这也是一种幸福。 …… 每个人都无一例外的渴望幸福。不同的人有不同的感受,其实,幸福就是那种能在平凡中寻找欢乐、能在困境中找到自信的一种心境。同学们,幸福其实很简单,就在我们的身边,触手可及。用心去认真地品味吧,它一直未曾离开我们身边! 感悟幸福650字2 有的人认为幸福就是腰缠万贯,有的人认为幸福就是找到意中人,“采菊东篱下,悠然见南山”是陶渊明对邪恶幸福,“从明天起做一个幸福人,喂马、劈柴、周游世界。从明天起,关心蔬菜和粮食,我有一所房子,面朝大海,春暖花开。”这是海子的幸福。一千种人就有一千种对幸福的理解。 我对幸福的理解就是幸福使简单而平凡的,是无处不在的! 我的牙疼得奇怪而顽强不是这颗牙疼就是那颗牙疼;不是吃冷的疼就是吃热 关于以幸福为话题的作文800字记叙文5篇 ----WORD文档,下载后可编辑修改---- 下面是作者为各位家长学生收集整理的作文(日记、观后感等)范本,欢迎借鉴参考阅读,您的努力学习和创新是为了更美好的未来,欢迎下载! 以幸福为话题的作文800字记叙文1: 那是我生病后的第三天,妈妈从早上五点就起来为我准备早点。她蹑手蹑脚地走着“针步”,下楼煮早点,“啪”的一声,妈妈打开了煤气。在拿肉丝,打鸡蛋的她全然不知我正躲在楼梯口“监视”着她的一举一动。不一会儿,蛋炒好了。 她开始切肉丝,一不小心,妈妈的手指切破皮了,鲜血正一滴一滴地流下来,为了不影响我的睡眠,她把手指放在嘴里吸了一下,坚持把剩下的肉丝切完。 此时的我,心中犹如打翻了五味瓶,眼里的泪像断了线的珍珠般掉了下来,我再也忍不住了,一个劲地冲到妈妈面前,她赶紧把手背了过去,生怕让我知道了什么。 她吃惊地问我:“妈妈太吵了,吵到你了?”“不,不,没有”她见我这么早起来就让我再回去补个觉。我关心地问:“妈,你的手没事吧?”她吱唔着说:“没事,擦破点皮,不碍事!”我仔细地帮她清洗了伤口,贴了一片创可贴。 吃饭时,妈妈一直地往我碗里夹肉,“孩子,病刚好,多吃点!”可是我见她始终都没吃一块肉。我也夹了两块放在她的碗里。“儿子懂事了,你自己快点吃吧!补身体要紧!”我冲她点点头笑了笑,“嗯。” 这就是幸福,一份简简单单的幸福!我祈祷这幸福能伴我成长。 以幸福为话题的作文800字记叙文2: 在我眼中,成长就是记录我们长大过程中一点一滴的小事情的,而幸福就在这点点滴滴中。 在我的成长记忆中,永不磨灭的是2017年11月的一天。妈妈要去云南,妈妈早上四点半要到指定地点集合,这么早,妈妈要两三点就起来,可是最近我咳嗽比较严重,所以天天给我煮萝卜汤喝。 “叮铃铃,叮铃铃”闹钟叫了起来,把我从睡梦中吵醒,一醒来,去找妈妈, 谈如何尊重人尊重他人,我们赢得友谊;尊重他人,我们收获真诚;尊重他人,我们自己也 获得尊重;相互尊重,我们的社会才会更加和谐. ——题记 尊重是对他人的肯定,是对对方的友好与宽容。它是友谊的润滑剂,它是和谐的调节器, 它是我们须臾不可脱离的清新空气。“主席敬酒,岂敢岂敢?”“尊老敬贤,应该应该!”共和 国领袖对自己老师虚怀若谷,这是尊重;面对许光平女士,共和国总理大方的叫了一 声“婶婶”,这种和蔼可亲也是尊重。 尊重不仅会让人心情愉悦呼吸平顺,还可以改变陌生或尖锐的关系,廉颇和蔺相如便是 如此。将相和故事千古流芳:廉颇对蔺相如不满,处处使难,但蔺相如心怀大局,对廉颇相 当的尊重,最后也赢得了廉颇的真诚心,两人结为好友,共辅赵王,令强秦拿赵国一点办法 也没有。蔺相如与廉颇的互相尊重,令得将相和的故事千百年令无数后人膜拜。 现在,给大家举几个例子。在美国,一个颇有名望的富商在散步 时,遇到一个瘦弱的摆地摊卖旧书的年轻人,他缩着身子在寒风中啃着发霉的面包。富 商怜悯地将8美元塞到年轻人手中,头也不回地走了。没走多远,富商忽又返回,从地摊上 捡了两本旧书,并说:“对不起,我忘了取书。其实,您和我一样也是商人!”两年后,富商 应邀参加一个慈善募捐会时,一位年轻书商紧握着他的手,感激地说:“我一直以为我这一生 只有摆摊乞讨的命运,直到你亲口对我说,我和你一样都是商人,这才使我树立了自尊和自 信,从而创造了今天的业绩??”不难想像,没有那一 句尊重鼓励的话,这位富商当初即使给年轻人再多钱,年轻人也断不会出现人生的巨变, 这就是尊重的力量啊 可见尊重的量是多吗大。大家是不是觉得一个故事不精彩,不够明确尊重的力量,那再 来看下一个故事吧! 一家国际知名的大企业,在中国进行招聘,招聘的职位是该公司在中国的首席代表。经 过了异常激烈的竞争后,有五名年轻人,从几千名应聘者中脱颖而出。最后的胜出者,将是 这五个人中的一位。最后的考试是一场面试,考官们都 作文话题素材之为人处世篇:尊重 思路 人与人之间只有互相尊重才能友好相处 要让别人尊重自己,首先自己得尊重自己 尊重能减少人与人之间的摩擦 尊重需要理解和宽容 尊重也应坚持原则 尊重能促进社会成员之间的沟通 尊重别人的劳动成果 尊重能巩固友谊 尊重会使合作更愉快 和谐的社会需要彼此间的尊重 名言 施与人,但不要使对方有受施的感觉。帮助人,但给予对方最高的尊重。这是助人的艺 术,也是仁爱的情操。———刘墉 卑己而尊人是不好的,尊己而卑人也是不好的。———徐特立 知道他自己尊严的人,他就完全不能尊重别人的尊严。———席勒 真正伟大的人是不压制人也不受人压制的。———纪伯伦 草木是靠着上天的雨露滋长的,但是它们也敢仰望穹苍。———莎士比亚 最新整理高中关于幸福的议论文800字范文3篇 范文一 什么是幸福?当我把一个棒棒糖递给六岁的邻居小妹妹时,她满足的笑容告诉我,这是她的幸福。当我轻轻地走过妹妹的写字台时,我瞥见埋在桌上的妹妹的僵硬的表情。我笑笑,走近,她抬头,水汪汪的眼睛望着我,似乎带着某种渴求。我说:出去玩吧!她笑了,蹦蹦跳跳地跑了出去。我诧异,这么真诚的笑。玩耍是她的幸福。 暑假到了,马上面临实习的哥哥回来了。可没过几天,就不见人影了,好容易盼他回来,暑假也结束了。他说他去了内蒙的好多地方。我关切的问他累吗?他说:累啊!随后又骄傲地说:“可是我学会了许多东西,我相信那对我以后的人生路是有帮助的。”我笑,大声地喊:哥,你是我的榜样。在他看来,他的暑假是充实的,他是幸福的! 夜幕降临,繁星点点。隔着一层帘,我看见常年劳作的父亲坐在那里,默默地吸着一支烟。灯光打在他的脸上,我看不清他的表情,只有那斑白的鬓角依稀可见。父亲真的老了,每天早出晚归来支撑这个家,他一定很累了。眼泪盈满了眼眶,最后还是不争气的流了下来……“咳、、咳、、”一阵剧烈的咳嗽声传来。我擦干眼泪,走到父亲旁边,父亲把那支烟熄灭,慈祥的笑笑,说:爸爸老了,不中用了。我说:没有啊!父女两开怀的笑了,笑声混着一个个烟圈飘向远方……我问父亲:爸,这么多年付出,这么多年劳作,你幸福吗?他坚定地告诉我,幸福!他说:“只要你们开开心心快快乐乐地成长,我做的一切都值得。”他又说:“霞,好好读书,爸爸赚钱供你上大学,我还没老呢,至少还能干XX年,20年……然后是一片寂静,我和父亲看着远方,那里有希望。 年迈的姥姥是家里的大长辈,他常常念叨:平安就是福。那也许是经历了人生的酸甜苦辣后的感悟吧!每逢新春,一大家人在姥姥家围着看电视时,那应该是她的幸福吧! 幸福是什么?它不是你一个人拥有一座豪宅,它是一家人在并不宽敞的屋子里谈笑风生。它不是你一个人有拥山珍海味,它是一家人和和乐乐的吃一些普通 感受幸福作文(15篇) 感受幸福作文第1篇: 幸福是什么?这是许多同学要问的问题。 很小的时候,我就明白钱能够买来一大盒巧克力;钱能够买来玩具汽车;钱能够买许多的美丽的洋娃娃;钱能够买来一个大楼…… 我以为有钱就是幸福。 倡我错了,钱虽然能够买来一屋子巧克力,但买了甜蜜,钱虽然能买到房子,可是却买来家庭幸福;钱虽然能买来药,可是却买来健康,钱虽然能买来闹钟,可是买来时间……那时,我又明白了有钱必须幸福。 以前,我总是为了一条连衣裙而朝思暮想,盼望有一天能够穿上裙子,去放风筝。那时候,我以为拥有就是幸福。 最终有一天,妈妈给我买了这条连衣裙,我高兴的一宿都没有睡觉。可是几天的新鲜劲没有了,穿上裙子后,我并没有什么改变,依然是一个黄毛丫头。于是把它扔到箱子里。几个月后,我又把它翻出来,可是已经小了,穿下了。我又明白了,虽然裙子很美,但都是暂时的,完美的时光总是转瞬消失。 “幸福是什么?”我依然没有感受到。 几年后,我在街上看到了一对耄耋老人,他们虽然履蹒跚,可是互相搀扶,有时抬头看看天上的云卷云舒,有时望望西天如血的残阳,她们脸上洋溢着的是满足和幸福。 噢,我明白幸福就是真情。虽然他们很穷,可是他们很 相爱。他们彼此珍惜,从感叹世界对他们的公平。往往有的有钱人,他们虽然很有钱,可是他们并幸福,因为他们的心总是被金钱和权势所占据了,根本享受了这天伦之乐。 幸福其实很简单,就是和爸爸、妈妈吃一顿饭,和他在一齐聊聊天。 感受幸福作文第2篇: 夜,悄悄地打开了黑暗,散布着一如既往的宁静,天上的繁星披上了闪装,正对着我的眼,似乎害怕我听到它们之间的悄悄话。 知何时,甘寂寞的虫儿起劲地奏起了动听的乐曲,清凉的微风夹杂着泥土的芳香悄悄地将白天的烦闷与喧嚣赶跑。夜,显得更加宁静而诗意了。 静静的,左思,右想,就这样静静地坐在楼顶上,感受着夜馈赠我的美妙。就连天上偶尔飘过的云朵,也像是怕惊动了夜的宁静,如绒毛在平静水面滑过般,显得那么轻柔而迷人。 今夜独处在空旷的夜空下,感受着夜带给我的美妙,幸福惬意溢满于心。原先自我一向以来苦苦追寻的幸福其实就在自我的身边。 以往,有人努力打拼,渴望生活富裕来获得幸福,可一辈子的艰辛拼搏使自我逐渐沦为金钱的奴隶,苦苦追寻的幸福也越寻越远,最终留给自我的是岁月无情地染白的头发。其实,幸福并非是追寻能得到的,幸福是一种感受,仅有用心感受身边的一切,你就能发现,幸福无处在,譬如,管贫 尊重他人的写作素材 导读:——学生最需要礼貌 著名数学家陈景润回厦门大学参加 60 周年校庆,向欢迎的人们说的第一句话是:“我非常高兴回到母校,我常常怀念老师。”被人誉为“懂得人的价值”的著名经济学家、厦门大学老校长王亚南,曾经给予陈景润无微不至的关心和帮助。陈景润重返母校,首先拜访这位老校长。校庆的第三天,陈景润又出现在向“哥德巴赫猜想”进军的启蒙老师李文清教授家中,陈景润非常尊重和感激他。他还把最新发表的数学论文敬送李教授审阅,并在论文扉页上工工整整写了以下的字:“非常感谢老师的长期指导和培养——您的学生陈景润。”陈景润还拜访了方德植教授,方教授望着成就斐然而有礼貌的学生,心里暖暖的。 ——最需要尊重的人是老师 周恩来少年时在沈阳东关模范学校读书期间 , 受到进步教师高盘之的较大影响。他常用的笔名“翔宇”就是高先生为他取的。周恩来参加革命后不忘师恩 , 曾在延安答外国记者问时说:“少年时代我在沈阳读书 , 得山东高盘之先生教诲与鼓励 , 对我是个很大的 促进。” 停奏抗议的反思 ——没有礼仪就没有尊重 孔祥东是著名的钢琴演奏家。 1998 年 6 月 6 日晚,他在汕头 举办个人钢琴独奏音乐会。演出之前,节目主持人再三强调,场内观众不要随意走动,关掉 BP 机、手提电话。然而,演出的过程中,这种令人遗憾的场面却屡屡发生:场内观众随意走动, BP 机、手提电话响声不绝,致使孔祥东情绪大受干扰。这种情况,在演奏舒曼作品时更甚。孔祥东只好停止演奏,静等剧场安静。然而,观众还误以为孔祥东是在渴望掌声,便报以雷鸣般的掌声。这件事,令孔祥东啼笑皆非。演出结束后,孔祥东说:有个 BP 机至少响了 8 次,观众在第一排来回走动,所以他只得以停奏抗议。 “礼遇”的动力 ——尊重可以让人奋发 日本的东芝公司是一家著名的大型企业,创业已经有 90 多年的历史,拥有员工 8 万多人。不过,东芝公司也曾一度陷入困境,土光敏夫就是在这个时候出任董事长的。他决心振兴企业,而秘密武器之一就是“礼遇”部属。身为偌大一个公司的董事长,他毫无架子,经常不带秘书,一个人步行到工厂车间与工人聊天,听取他们的意见。更妙的是,他常常提着酒瓶去慰劳职工,与他们共饮。对此,员工们开始都感到很吃惊,不知所措。渐渐地,员工们都愿意和他亲近,他赢得了公司上下的好评。他们认为,土光董事长和蔼可亲,有人情味,我们更应该努力,竭力效忠。因此,土光上任不久,公司的效益就大力提高,两年内就把亏损严重、日暮途穷的公司重新支撑起来,使东芝成为日本最优秀的公司之一。可见,礼,不仅是调节领导层之间关 感悟幸福(作文)(20篇) 篇一篇的乐章组成的,喜、怒、哀、乐。每一个人都自我丰富多彩的生活,我也自我的生活。我原本以为,吃可口的牛排,打电脑游戏,和朋友开心玩乐就是幸福。可是,我错了,幸福并不仅仅如此。 记得一次,我放学回到家里放下书包就拿起一包饼干来吃。吃着吃着,突然我觉得牙齿痛了起来,并且越来越痛,痛得我连饼干也咬不动了。我放下饼干,连忙去拿了一面镜子来看。原先这又是那一颗虫牙在“作怪”。“哎哟哟,哎哟哟,痛死我了”我不停地说着。渐渐地,那牙疼得越来越厉害,疼得我坐立不安,直打滚。之后在妈妈的陪伴下去了医院,治好了那颗虫牙。跨出医院大门时,我觉得心境出奇的好,天空格外的蓝,路边的樟树异常的绿,看什么都顺眼,才猛然一悟,幸福是简单而平凡的,身体健康就是一种幸福! 这学期我发现我的英语退步了,我决定要把这门功课学好,于是,我每一天回家做完作业后,都抽出半小时时间复习英语,在课上也听得异常认真,一遇到不懂的题目主动请教教师。经过一段时间的努力,最终,在上次考试的时候,我考了97分。妈妈表扬了我,我心里美滋滋的。我明白了经过自我的努力享受到成功的喜悦,这也是一种幸福。 每个人都无一例外的渴望幸福。不一样的人不一样的感受,其实,幸福就是那种能在平凡中寻找欢乐、能在困境中找到自信的一种心境。同学们,幸福其实很简单,就在我们的身边,触手可及。用心去认真地品味吧,它一向未曾离开我们身边! 感悟幸福(作文) 第8篇: 幸福无时没,幸福无处不在。儿时,你的幸福就是当你跌倒失声痛苦时,母亲抱起你,拍打着你背时的那句“别哭。”;成年时,你的幸福就是在你结婚时你身旁的那位拉起你的手问你的那句“你愿意吗”;老年时,你的幸福就是在椅子背后孙子的那句“奶奶我帮你敲背!”。家庭中,幸福是一家子的和乐融融;学校里,幸福是同学间的互相竞争;赛场上,幸福是队友间的默契配合。 然而,幸福不全然是唾手可得的,想不付出任何代价而得到幸福,那是神话。张志新说;“苦换来的是知识真理,坚持真理,就应自觉的欣然理解痛苦,那时,也仅那时,痛苦才将化为幸福。”仅心身遭受过痛苦的洗涤,幸福才会来到,痛得越深,苦得越彻,幸福得就越甜。鲁迅说:“穿掘到灵魂的深处,使人受了精神的苦刑而得到的创伤,又即从这得伤和养伤的愈合中,得到苦的涤除,而上了苏生的路。”从而得到了幸福的真谛。拉罗什夫科说过:“幸福后面是灾祸,灾祸后面是幸福。”你想成为幸福的人吗愿你首先吃得 尊重_议论文素材 "礼遇"的动力 --尊重可以让人奋发 日本的东芝公司是一家著名的大型企业,创业已经有90 多年的历史,拥有员工8 万多人。不过,东芝公司也曾一度陷入困境,土光敏夫就是在这个时候出任董事长的。他决心振兴企业,而秘密武器之一就是"礼遇"部属。身为偌大一个公司的董事长,他毫无架子,经常不带秘书,一个人步行到工厂车间与工人聊天,听取他们的意见。更妙的是,他常常提着酒瓶去慰劳职工,与他们共饮。对此,员工们开始都感到很吃惊,不知所措。渐渐地,员工们都愿意和他亲近,他赢得了公司上下的好评。他们认为,土光董事长和蔼可亲,有人情味,我们更应该努力,竭力效忠。因此,土光上任不久,公司的效益就大力提高,两年内就把亏损严重、日暮途穷的公司重新支撑起来,使东芝成为日本最优秀的公司之一。可见,礼,不仅是调节领导层之间关系的纽带,也是调节上下级之间关系,甚至和一线工人之间关系的纽带。世界知识产权日 --尊重知识 在2000 年10 月召开的世界知识产权组织第35 届成员国大会上,我国提议将 4 月26 日定为"世界知识产权日"。这个提案经世界知识产权组织成员国大会得到了确定。2001 年4 月26 日成为第一个"世界知识产权日"。这是我国尊重知识的具体表现。 屠格涅夫与乞丐 --尊重比金钱更重要 俄罗斯文豪屠格涅夫一日在镇上散步,路边有一个乞丐伸手向他讨钱。他很想有所施与,从口袋掏钱时才知道没有带钱袋。见乞丐的手伸得高高地等着,屠格涅夫面有愧色,只好握着乞丐的手说:"对不起,我忘了带钱出来。"乞丐笑了,含泪说:"不,我宁愿接受您的握手。" 孙中山尊重护士 --尊重不分社会地位 有一天,孙中山先生病了,住院治疗。当时,孙中山已是大总统、大元帅了。但是,他对医务人员很尊重,对他们讲话很谦逊。平时,无论是早晨或是晚间,每当接到护士送来的药品,他总是微笑着说声"谢谢您",敬诚之意溢于言辞。 1925 年孙中山患肝癌,弥留之际,当一位护理人员为他搬掉炕桌时,孙中山先生安详地望着她,慈祥地说:"谢谢您,您的工作太辛苦了,过后您应该好好休息休息,这阵子您太辛苦了! "听了这话,在场的人都泣不成声。 毛泽东敬酒 --敬老尊贤是一种美德 1959 年6 月25 日,毛泽东回到离别30 多年的故乡韶山后,请韶山老人毛禹珠来吃饭,并特地向他敬酒。毛禹珠老人说:"主席敬酒,岂敢岂敢! "毛泽东接着说:"敬老尊贤,应该应该。" 周恩来不穿拖鞋接待外宾 --衣着整齐体现对人的尊重 周恩来晚年病得很重,由于工作的需要,他还要经常接待外宾。后来,他病得连脚都肿起来了,原先的皮鞋、布鞋都不能穿,他只能穿着拖鞋走路,可是,有些重要的外事活动,他还是坚持参加。他身边的工作人员出于对总理的爱护和关心,对他说:"您就穿着拖鞋接待外 关于尊重的论点和论据素材 关于尊重的论点 1.尊重需要理解和宽容。 2.尊重也应该坚持原则。 3.尊重知识是社会进步的表现。 4.尊重别人就要尊重别人的劳动。 5.尊重人才是社会发展的需要。 6.人与人之间需要相互尊重。 7.只有尊重别人才会受到别人的尊重。 8.尊重能促进人与人之间的沟通。 9.我们应该养成尊重他人的习惯。 10.对人尊重,常会产生意想不到的善果。 关于尊重的名言 1.仁者必敬人。《荀子》 2.忍辱偷生的人决不会受人尊重。高乃依 3.尊重别人的人不应该谈自己。高尔基 4.尊重别人,才能让人尊敬。笛卡尔 5.谁自尊,谁就会得到尊重。巴尔扎克 6.君子贵人而贱己,先人而后己。《礼记》 7.卑己而尊人是不好的,尊己而卑人也是不好的。徐特立 8.对人不尊敬,首先就是对自己的不尊敬。惠特曼 9.为人粗鲁意味着忘记了自己的尊严。车尔尼雪夫斯基 10.对人不尊敬的人,首先就是对自己不尊重。陀思妥耶夫斯基 11.对于应尊重的事物,我们应当或是缄默不语,或是大加称颂。尼采 12.尊重老师是我们中华民族的传统美德,我们每一个人都不应该忘记。xx 13.尊重劳动、尊重知识、尊重人才、尊重创造。《xx 大报告》 14.对别人的意见要表示尊重。千万别说:你错了。卡耐基 15.尊重人才,培养人才,是通用电器长久不败的法宝。杰克韦尔奇 16.君子之于人也,当于有过中求无过,不当于无过中求有过。程颐 17.施与人,但不要使对方有受施的感觉。帮助人,但给予对方最高的尊重。这是助人的艺术,也是仁爱的情操。刘墉 18.要尊重每一个人,不论他是何等的卑微与可笑。要记住活在每个人身上的是和你我相同的性灵。叔本华 19.要喜欢我们所不尊重的人是很难的;但要喜欢 感受幸福作文范文5篇 幸福是一种心灵的振颤。它像会倾听音乐的耳朵一样,需要不断地训练。下面给大家了感受幸福范文5篇,仅供参考。 我曾经填了一份读者反馈表,得了个二等奖,奖品是三百元钱。拿着那张三百元汇款单回家,我却不大开心。在路上,我想起了从前看过的一部电影,片名好像是《小鞋子》。 主人公小阿里在参加比赛时,一心只想跑第三名,以拿到梦寐以求的奖品——一双小鞋子。他想让妹妹穿着它,而不是光着脚去上学。 他被别人推倒了又爬起来再跑,情急之下,竟然冲到了第一名。 老师欣喜若狂地祝贺他,主办单位安排大官和他合影,阿里却难过地流下了眼泪。 尽管冠军有着很高的荣誉,能得到更为丰富的奖品,但那不是阿里最需要的,他需要那双并不漂亮的小鞋子。结果,他不仅没有得到,反而连仅有的一双鞋子也跑坏了。 在那个时候,我们或许可以这样解释幸福:幸福就是得到你急需的东西。 一个吃斋念佛的饥饿的人,面对着驰名中外的北京烤鸭,是不会有幸福感的,因为那不是他需要的,他急需的,可能只是两个馒头,一盘素炒豆芽而已;独自住在乡下的老人,总是能收到城里儿子寄来的钱物,很让乡亲们羡慕,但老人最想要的不是这些,而是儿孙膝下承欢的天伦之乐;在炎炎烈日下赶路的人,急需的是一片树阴,一阵凉风;一把扇子,一眼清凉的泉水,而不是一件名贵的貂皮大衣;大海是富饶的,它哺育了各种各样的生物,珍藏着无数的宝藏和财富。但在海上迷航的人,急需的却是普普通通的淡水。 聪明的你,可能已经猜到, ___不开心了。是的,我不需要三百元钱,钱我家里人会给我。我原本只想得个三等奖,三等奖的奖品是一百元钱。我想留为己用,不想让家里人知道。尽管这样做很不合理,但我还是想,因为我想。 幸福,是团聚;是分享快乐;是帮助他人;是朋友团结互助;是一个美梦;是……幸福无处不在,只要用心体会,就能感受到幸福的存在。 关于学会尊重的高中作文1000字以上 尊重是一杯清茶,只有真正懂它的人才能忽略它的寡淡,品出它深处的热烈。下面橙子为大家搜集整理有关学会尊重的高中作文,希望可以帮助到大家! 高中作文学会尊重曾经听说这样一个故事: 一位商人看到一个衣衫破烂的铅笔推销员,顿生一股怜悯之情。他不假思索地将10元钱塞到卖铅笔人的手中,然后头也不回地走开了。走了没几步,他忽然觉得这样做不妥,于是连忙返回来,并抱歉地解释说自己忘了取笔,希望不要介意。最后,他郑重其事地说:“您和我一样,都是商人。” 一年之后,在一个商贾云集、热烈隆重的社交场合,一位西装革履、风度翩翩的推销商迎上这位商人,不无感激地自我介绍道:“您可能早已忘记我了,而我也不知道您的名字,但我永远不会忘记您。您就是那位重新给了我自尊和自信的人。我一直觉得自己是个推销铅笔的乞丐,直到您亲口对我说,我和您一样都是商人为止。” 没想到商人这么—句简简单单的话,竟使一个不无自卑的人顿然树立起自尊,使—个处境窘迫的人重新找回了自信。正是有了这种自尊与自信,才使他看到了自己的价值和优势,终于通过努力获得了成功。不难想象,倘若当初没有那么—句尊重鼓励的话,纵然给他几千元也无济于事,断不会出现从自认乞丐到自信自强的巨变,这就是尊1 / 7 重,这就是尊重的力量! 尊重,是—种修养,一种品格,一种对别人不卑不亢的平等相待,一种对他人人格与价值的的充分肯定。任何人都不可能尽善尽美,完美无缺,我们没有理由以高山仰止的目光审视别人,也没有资格用不屑一顾的神情去嘲笑他人。假如别人在某些方面不如自己,我们不能用傲慢和不敬去伤害别人的自尊;假如自己在有些地方不如他人,我们不必以自卑或嫉妒去代替理应有的尊重。一个真正懂得尊重别人的人,必然会以平等的心态、平常的心情、平静的心境,去面对所有事业上的强者与弱者、所有生活中的幸运者与不幸者。 尊重是一缕春风,一泓清泉,一颗给人温暖的舒心丸,一剂催人奋进的强心剂。它常常与真诚、谦逊、宽容、赞赏、善良、友爱相得益彰,与虚伪、狂妄、苛刻、嘲讽、凶恶、势利水火不容。给成功的人以尊重,表明了自己对别人成功的敬佩、赞美与追求;表明了自己对别人失败后的同情、安慰与鼓励。只有要尊重在,就有人间的真情在,就有未来的希望在,就有成功后的继续奋进,就有失败后的东山再起。 尊重不是盲目的崇拜,更不是肉麻的吹捧;不是没有原则的廉价逢迎,更不是没有自卑的低三下四。懂得了尊重别人的重要,并不等于学会了如何尊重别人,从这个意义上说,尊重他人也是一门学问,学会了尊重他人,就学会了尊重自己,也就学会和掌握了人生的一大要义。 2 / 7 感受幸福作文600字叙事感受幸福作文600字初二 对于我们来说,什么是幸福?幸福就是一家人整整齐齐的坐在一起吃一顿饭,幸福就是自己在乎的人没病没痛,健健康康的.....今天小编和同学们分享几篇关于感受幸福的作文,希望帮到有需要的同学们。感受幸福作文600字【1】有人说,幸福是一种感觉,幸福是一种心境,幸福是一种体验,幸福,更是一种品格。忧伤时,贴心好友的一个拥抱是幸福;思乡时,电话那头响起的家人声音是幸福;听着音乐,啜着咖啡,悠然自在地写点感想是幸福……那么,朋友,你感受到了幸福了吗?幸福,有些人看来很渺远,有些人看来却近在咫尺。其实,世界并不缺少幸福,而是缺少发现幸福的眼睛。“猫吃鱼,狗吃肉,奥特曼打小怪兽”各求所需,心满意足,这,就是所谓的“幸福”。年轻的父母在逗弄牙牙学语的孩童;亲密爱人在携手漫步;父母端上热腾腾的饭菜;孩子熟睡后嘴角的微笑……这点点滴滴不都是幸福的剪影吗?幸福无处不在……有一次,妈妈烧了红烧鱼,刚要端上桌,我贪婪的目光就随着鱼的移动而移动。妈妈忙把好吃的鱼肚子夹给我,说:“来,吃吧!”“咦?你不是最爱吃这个吗?”我反问道。“现在不爱吃了,你吃吧!”妈妈说道。我拗不过妈妈,只好吃了。刹时间,幸福的感觉洋溢全身。只有在菜里放入了爱,才能让人感受到幸福。平凡的红烧鱼,不平凡的味道,不平凡的爱……幸福,往往是一个瞬间,只有有爱的人,才能发现幸福,感受幸福。朋友,感受你身边的幸福吧!幸福,无处不在……感受幸福作文600字【2】 人生若是一夜星空,幸福便是那耀眼的星星,琳琅满目。当你仔细观察时,你会发现,每一颗都独一无二。——题记 抬头仰望星空,看着那琳琅满目的星星,每一颗都独一无二。当我的眼神扫到最亮的那一颗时,我的思绪立马随着那颗星星穿越到过去。“神经病啊你,我都说过几百遍几千吧几万遍了,我的东西你别碰,你为什么就是讲不通听?好了,现在玻璃球打碎了,可以了吧?你舒服了吧?”扯着嗓子喊的是一个七岁的小女孩,她的对面站着一个五六岁的小女孩,那小女孩有着圆圆的脑袋,一头乌黑发亮的头发下有一双水灵灵的大眼睛,高挺的鼻梁下一张樱桃小嘴,模样甚是可爱。只是那眼眶湿润着,泪水如断了线的珍珠,不断地往外涌。她的小嘴蠕动了好几次,却始终没有说话,小女孩颤颤巍巍地向前走去,用颤抖的小手慢慢地拾起地下的玻璃碎片,转身离开。第二天清晨,当那个七岁的小女孩睁开眼时,就看到桌上有一个玻璃球和小纸条。纸条上写着歪歪扭扭的小字:姐姐对不起,我不是故意的,我只是觉得玻璃球很漂亮。现在我又帮你重新买了一个一模一样的玻璃球,希望姐姐不要生气了。童年的易满足心,那个小女孩果真没有生气了,她和那个小妹妹和好如初了。但偶然的一天,她却发现,玻璃球不是小妹妹打破的……原来是邻居的两岁小弟弟,看到那个玻璃球很漂亮,在伸手拿它时,因为太滑,玻璃球滚了下来……于是,她拿着自己最爱的零食和棒棒糖去和小妹妹道歉。没想到妹妹却如小大人一般说:“都过去了,你依旧是我的好姐姐,我永远是你的调皮妹妹……”回想到这里,我的眼眶早已红润。那个七岁的小女孩不就是我吗?那个小妹妹不就是自己的妹妹吗?顿时明白了妹妹的用意,顿时发现:幸福就像空气,无时无刻萦绕在我们身边,只是我们未曾发觉罢了。现在的我发觉了,也感受到了。人生若是一夜星空,幸福便是那耀眼的星星,琳琅满目。当你仔细观察时,你会发现,每一颗都独一无二。感受幸福作文600字【3】 什么是幸福?幸福是空气,它普遍的你随处都可以感受到它的存在;幸福是小鸟,它调皮的你怎么也抓不住它;幸福又是一杯水,平平淡淡才是真。幸福无时没有,幸福无处不在。儿时,你的幸福就是当你跌倒失声痛苦时,母亲抱起你,拍打着你背时的那句“别哭。”;成年时,你的幸福就是在你结婚时你身旁的那位拉起你的手问你的那句“你愿意吗?”;老年时,你的幸福就是在椅子背后孙子的那句“奶奶我帮你敲背!”。家庭中,幸福是一家子的和乐融融;学校里,幸福是同学间的互相竞争;赛场上,幸福是队友间的默契配合。然而,幸福不全然是唾手可得的,想不付出任何代价而得到幸福,那是神话。张x新说;“苦换来的是知识真理,坚持真理,就应自觉的欣然接受痛苦,那时,也只有那时,痛苦才将化为幸福。” 感悟幸福作文(精选10篇) 在日常生活或是工作学习中,大家都经常接触到作文吧,作文是由文字组成,经过人的思想考虑,通过语言组织来表达一个主题意义的文体。那么,怎么去写作文呢?以下是小编收集整理的感悟幸福作文(精选10篇),仅供参考,希望能够帮助到大家。 感悟幸福作文1 幸福是“临行密密缝,意恐迟迟归”的牵挂;幸福是“夜来风雨声,花落知多少”的恬谈;幸福是“先天下之忧而忧,后天下之乐而乐”的胸怀。 记得那是一个宁静的夜晚,家家户户家家户户都关了灯,可我家的灯却依旧工作着,因为我的作业还没有写完,懊恼的我使劲的捶打着桌子。妈妈听到了响声走了进来,亲切的说到:“怎么了乖女儿?”“都六点半了,我的作业还没有写完,我怎么不着急”,我气急败坏的回答。妈妈看到我无助的状态安慰到“孩子不要着急,妈妈会在这一直陪着你,直到你写完作业……”此刻我忽然感觉到,其实有母亲的陪伴就是我最大的幸福! 还有一次,我和同学们一起在小区的花园里玩,正当我们玩游戏玩的不亦乐乎的时候,我被重重的摔了一跤,腿被摔破了。当时情不自禁的哭了起来,同学们听到我的哭声纷纷赶来,大家纷纷帮忙把我搀扶回家里。那时我又深深的感觉到,来自朋友的关心其实也是一种幸福。 记得那是一个风雨交加的下午,由于我的粗心来学的时候忘记了带雨伞。放学后我只能站在教室的窗口默默的看着外面的漂泊大雨……忽然,一个高大而熟悉的身影出现在我的眼前,忽然感觉双眼酸涩:“爸爸……”我一下子扑倒了爸爸的怀里,父亲的爱是无言的,这也正是我幸福的源泉! 幸福就是有母亲的陪伴,朋友的关怀,父亲的关爱!每个人都向往幸福追求幸福,其实幸福就是一种感觉,是一种心态,它源于你对事与物的的追求与理解! 感悟幸福作文2 著名作家海伦凯勒曾说过:“我一直在哭,一直在哭,哭我没有鞋穿,直到有一天,我知道有人没有脚…我由此知道了幸福的真正内涵。”幸福是什么? 许多年前,当人们提出这个问题时,个个都两目相对,哑口无言。是啊,幸福是什么?幸福是那禾苗晃动稚嫩的身躯,昂首向天际发出的无声的诉说吗?幸福是蚕宝宝咀嚼着香脆的桑叶奏出的“沙沙沙沙”的乐曲吗?幸福是雏燕展翼穿过鹅黄的柳梢剪出的一片春色朝向母亲的回眸吗?幸福是梅花“已是 2015年高考作文素材:关于文明的作文素材 高考作文素材点拨及运用思路:文明 一、素材链接: 言语类 (一)名人名言 1.礼仪的目的与作用本在使得本来的顽梗变柔顺,使人们的气质变温和,使他尊重别人,和别人合得来。约翰?洛克 2.善气迎人,亲如弟兄;恶气迎人,害于戈兵。管仲 3.天下有大勇者,猝然临之而不惊,不故加之而不怒。苏轼 4.我们应该注意自己不用言语去伤害别的同志,但是,当别人用语言来伤害自己的时候,也应该受得起。刘少奇 5.礼貌使人类共处的金钥匙。松苏内吉 6.讲话气势汹汹,未必就是言之有理。萨迪 7.火气甚大,容易引起愤怒底烦扰,是一种恶习而使心灵向着那不正当的事情,那是一时冲动而没有理性的行动。彼得.阿柏拉德 8.青年人应当不伤人,应当把个人所得的给予各人,应当避免虚伪与欺骗,应当显得恳挚悦人,这样学着去行正直。夸美纽斯 9.礼貌是儿童与青年所应该特别小心地养成习惯的第一件大事。约翰.洛克 10.不论你是一个男子还是一个女人,待人温和宽大才配得上人的名称。一个人的真 11.正的英勇果断,决不等于用拳头制止别人发言。萨迪 12.礼貌使有礼貌的人喜悦,也使那些授人以礼貌相待的人们喜悦。孟德斯鸠 13.坏事情一学就会,早年沾染的恶习,从此以后就会在所有的行为和举动中显现出来,不论是说话或行动上的毛病,三岁至老,六十不改。克雷洛夫 14.礼貌经常可以替代最高贵的感情。梅里美 15.礼貌是最容易做到的事,也是最珍贵的东西。冈察尔 16.脾气暴躁是人类较为卑劣的天性之一,人要是发脾气就等于在人类进步的阶梯上倒退了一步。达尔文 17.蜜蜂从花中啜蜜,离开时营营的道谢。浮夸的蝴蝶却相信花是应该向他道谢的。泰戈尔 感受幸福作文650字 第1篇感受幸福作文650字 什么是幸福?幸福是各种各样的,不同的人对幸福的感受也各不相同。假如我们不会说话,那就不能表达自己内心的想法,不能向朋友倾诉自己的喜悦与烦恼,那是一种不幸,反之就是一种幸福。假如我们听不见,就不能感受到一切美妙的声音,如叮叮咚咚的琴声,哗哗的流水声,以及父母、老师、同学的说话声,那也是一种不幸,反之也是一种幸福。假如我们看不见,就不能欣赏到这个大千世界形形色色的样子,看不见斑斓的色彩,更看不见那美丽的太阳和月亮……这也是一种不幸,反之,,也是一种幸福。父母爱着我,就是一种幸福,别人帮助我,是一种幸福,能吃到美味的食物,也是一种幸福,快乐地生活着,更是一种幸福。被人信任是一种莫大的幸福。我平时有个坏习惯,不喜欢洗脸,一般都要妈妈督促着才勉强去洗。可有一次,妈妈回家比较晚。她到家时,我们都已经在吃晚饭了,正吃得津津有味时,妈妈回来了,她的第一句话就是“你今天有没有洗过脸?”,我赶紧回答:“我洗过了”。妈妈半信半疑:“你到底洗过没有?”,并盯着我,脸上的表情十分严肃。她这样做,其实是测试我有没有说谎。于是我更加坚定并大声地说:“我真的洗过了!”这次,她不再问了,并且笑了,我知道她相信了我的话,这时,我真的感受到了一种从未有过的幸福!其实,幸福还有很多很多,如爷爷奶奶的宠爱,父母亲的关怀,亲戚朋友们的赞美,老师们的期盼,同学们的友爱…。.都值得我们去好好珍惜,让我们常怀一颗感恩的心,时刻感受生活中的幸福吧! 第2篇感受幸福作文650字 欧文曾说过,“人类的一切努力的目的在于获得幸福。幸福是什么?幸福是一种感觉,感动就是一种幸福。幸福就像作家毕淑敏说的:世上有预报台风的,有预报蝗虫的,有预报瘟疫的,有预报地震的,但没有人预报幸福,其实幸福和世界万物一样,有它的征兆。清晨,旭日东升,温柔的阳光洒向人间,抚摸着我的脸,心,感觉暖暖的。这,就是幸福的征兆。当我们在写作业时,一边写,一边贪婪地记下知识的结晶。这时,笔尖沙沙的响声,就是幸福的征兆。走在小河边,两岸的杨柳随风摆动,鱼儿和鹅卵石正在编制着充满活力的动态画面。这时,小河潺潺的流水声就是幸福的征兆。幸福的感觉是美好的,幸福的味道是甜美的。只不过它比玫瑰更芬芳,比棒棒糖更甜蜜,比巧克力更浓郁,比咖啡更香醇......幸福是什么?“幸福是贫困中相濡以沫的一块糕饼,患难中心心相印的一个眼神,父亲一次粗糙的抚摸,女友一个温馨的字条......这像一粒粒缀在旧绸子上的红宝石,在凄凉中愈发熠熠生辉。”幸福在哪里?当我们与家人一起散步时,幸福就在那温馨的眼神里;当我们和朋友们一起玩耍时,幸福就在那灿烂的笑容里;当我们与同学在一起探讨难题时,幸福就在那激烈的争辩里;当我们想象着自己的未来时,幸福就在那美好的憧憬里。幸福有时不一定是甜的,快乐有时也不一定就是幸福。快乐只是表面的开心,而幸福是发自内心的快乐和欣慰。因此,只要你是幸福的,那你一定是快乐的。让我们一起追求幸福吧!在生活中快乐成长,在成长中感受幸福。 第3篇感受幸福作文650字 幸福是什么?幸福对于每个人,都是不一样的!其实幸福可以很小,很简单,它时刻陪伴在我们的身边,也许是因为人们平时没有去在意,身边的那些本可以让人暖心的普通点滴,只要我们好好的去感受幸福! 幸福就好比如这样:天悄悄拉下夜幕,同学们渐渐散了,不知什么时候,课室里只剩下我和她,她的画,也终于画好了。而我还是差一些才能搞定,她便开始收拾起来,时间更加紧迫,因此,我的心更加紧张,一滴晶莹的汗滴在了画纸上,我觉得实在画不下去了:小姨妈(外号),你觉得我的画还有有救吗!她凑过脑袋:来,我看看,当然有啦,快画吧!我看她望了望校门口,她一定是在找寻来接她回家的爸爸,我看看空空的课室:小姨妈,很晚尊重的素材
那一刻我感受到了幸福_初中作文
关于我的幸福作文八篇汇总
小学生作文《感悟幸福》范文五篇汇总
关于以幸福为话题的作文800字记叙文5篇
尊重议论文
最新整理高中关于幸福的议论文800字范文3篇
感受幸福作文(15篇)
尊重他人的写作素材
感悟幸福(作文)(20篇)
尊重_议论文素材
关于尊重的论点和论据素材
感受幸福作文范文5篇
关于学会尊重的高中作文1000字以上_作文素材
感受幸福作文600字叙事 感受幸福作文600字初二
感悟幸福作文(精选10篇)
高考作文素材关于文明的作文素材
感受幸福作文650字