Mcm2014美赛优秀论文
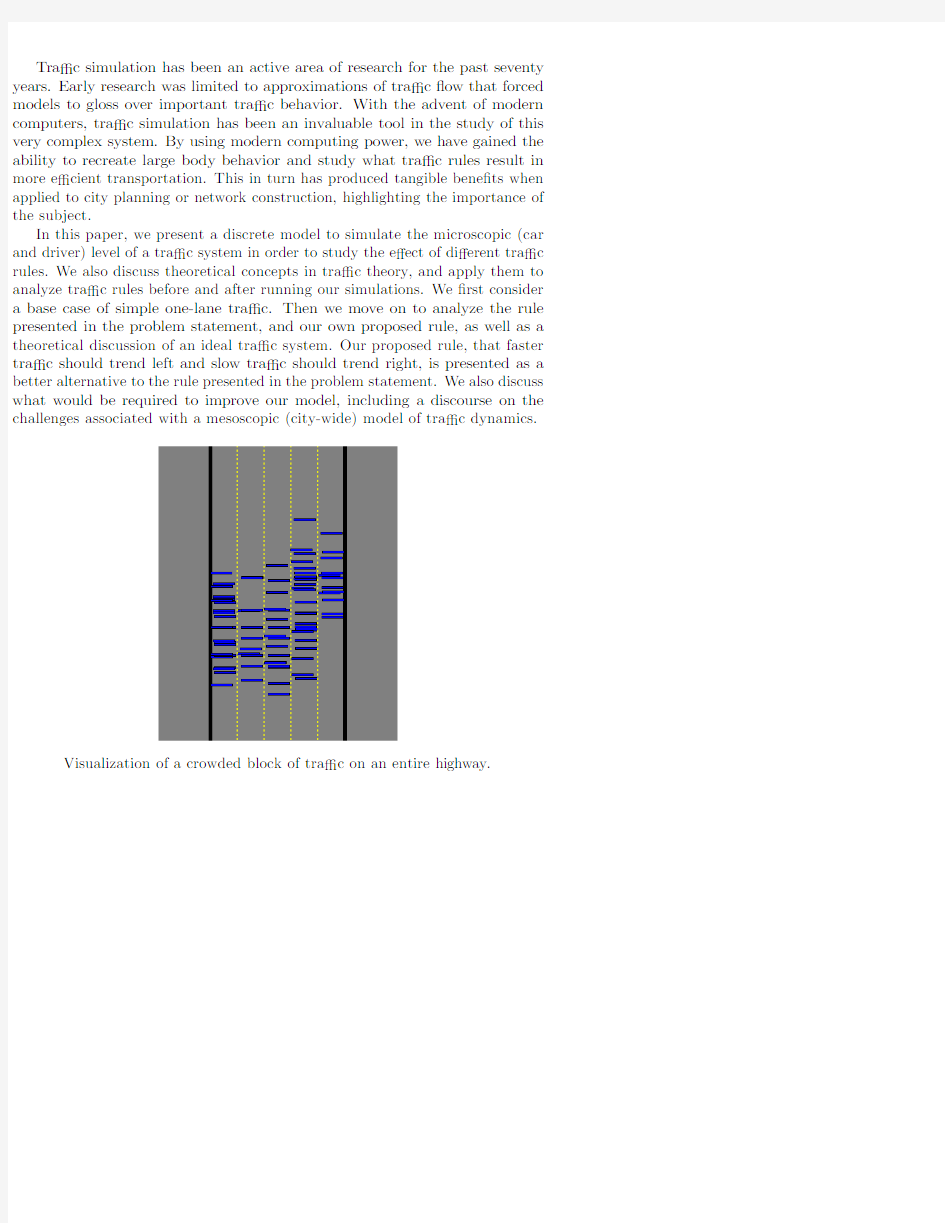

Control#29221 Tra c simulation has been an active area of research for the past seventy years.Early research was limited to approximations of tra c?ow that forced models to gloss over important tra c behavior.With the advent of modern computers,tra c simulation has been an invaluable tool in the study of this very complex system.By using modern computing power,we have gained the ability to recreate large body behavior and study what tra c rules result in more e cient transportation.This in turn has produced tangible bene?ts when applied to city planning or network construction,highlighting the importance of the subject.
In this paper,we present a discrete model to simulate the microscopic(car and driver)level of a tra c system in order to study the e?ect of di?erent tra c rules.We also discuss theoretical concepts in tra c theory,and apply them to analyze tra c rules before and after running our simulations.We?rst consider a base case of simple one-lane tra c.Then we move on to analyze the rule presented in the problem statement,and our own proposed rule,as well as a theoretical discussion of an ideal tra c system.Our proposed rule,that faster tra c should trend left and slow tra c should trend right,is presented as a better alternative to the rule presented in the problem statement.We also discuss what would be required to improve our model,including a discourse on the challenges associated with a mesoscopic(city-wide)model of tra c dynamics.
Visualization of a crowded block of tra c on an entire highway.
Beating the Tra c:An Analysis of Alternative
Tra c Rules
Control#29221
February10,2014
Page1of13Control#29221
Contents
1Introduction2
1.1Outline of Approach (2)
1.2Assumptions (2)
2Background3
2.1De?nitions (3)
2.2Introduction to our Model (4)
3Analysis of the Standard Maneuver6
3.1Theoretical Approach (6)
3.2Computational Approach (6)
4Analysis of a New Rule8
4.1Theoretical Approach (8)
4.2Computational Approach (8)
5Modi?cations and Extensions10
5.1Driving on the Left Side (10)
5.2Safety Trade-o? (10)
5.3Varied Speed Limits (11)
5.4An Ideal System (11)
6Conclusion11 7References13
Page2of13Control#29221
1Introduction
1.1Outline of Approach
In this paper we will present a discrete model for small scale tra c?ow.We de?ne small scale tra c to be tra c where the signi?cant dynamics are determined by actions of individual drivers,as opposed to large scale where one might just look at di?erential equations governing the?ow,with little or no interaction from individuals who make up that?ow.We present some tra c theory de?nitions and construct a discretized model of a highway under varying tra c load and varying rules for how drivers behave.The basic structure of each model is the same,we have a road which has been discretized into4-foot sections,each car will take up4of these sections.Each road has a varying number of lanes,from1 to5.Cars are placed in positions in each lane and we then take time steps of 1second.In each time step every driver will make a choice about whether to switch lanes or to maintain,decrease,or increase their velocity.When sequenced together we have information about positions and velocities unique to each car in our model for the entire time we run the model.We will then analyze these results and compare di?erent tra c rules in order to get a better understanding of why certain rules are more e cient at allowing tra c?ow,while highlighting the?aws in others.
1.2Assumptions
?In our models we assume that the speed limit is approximately 71miles per hour.
This corresponds to a velocity of26units per time step as we use4foot units and1second time steps.Highway speeds vary from55to85miles per hour on average depending on population and road size.In a sparsely populated area with a reasonable sized highway75miles per hour seems to be a good average.
?We assume that no driver speeds,
For simplicity sake,this is of course an untrue assumption,but when determining the usefulness of a law,we should assume that all other laws relating are followed,otherwise our analysis would be of the system of laws, not an objective evaluation of any single law.In order to not lock cars out of being able to merge,we allowed drivers to merge even if they would be bumper to bumper.This helps to simulate the fact that in reality,drivers will often allow others to merge,while our model isn’t that interactive.?We assume that drivers are making decisions according to our rules and reacting within one second.
We assume that the maximum breaking speed is 28ft/s2,and the maxi-mum acceleration is4ft/s2,this is based on information from the United States Department of Transportation.The?nal assumption we made was
Page3of13Control#29221 that if two cars are in a collision then they are removed from the road.In reality,they would probably pull over,but in our model they are instantly removed from the simulation to reduce complexity.
2Background
2.1De?nitions
We will start by de?ning several statistics from tra c theory following the standards introduced in[4].The?rst important quantity is the“?ow”of a highway.Intuitively we want to talk about the amount of tra c that goes across a highway.To calculate the?ow,we imagine taking a tiny slice of road across the lanes of our highway.Then,we start a stopwatch wait and count the number of cars that travel over this slice.Once our stopwatch hits a predetermined value (called?in our paper),we stop counting the cars.The ratio of cars crossed to time elapsed is our value for q,the“?ow”.However,our tra c model is discrete in nature,so both our“tiny”slice of road and our“tiny”slice in time are actually sizable.This means that in order to achieve good values for q,we must average the?ow over a time period much longer than our discretization.
There is also another critical quantity we need to introduce from tra c theory, called the“space-averaged density”.The letter reserved for this is usually k, and this notation is used throughout the rest of our paper.k is the number of vehicles in a given strip of highway.Again,imagine placing a marker at some point x1,and another marker further down the road at some point x2.Then,at a particular time,we count the number of cars between the two markers.The ratio of counted cars to the distance of our markers is k.k is a quantity,like q, that makes sense in an averaged context.You could imagine moving the markers around and getting di?erent values for k due to the fact that cars are discrete, but this e?ect is diminished if we only consider large stretches of highway.
q and k can,and should,vary over a highway,especially if certain regions are heavier with tra c than others,such as a merging lane or an accident.To get an idea of how q changes as a function of k(the density of tra c),we need to average q over the highway.This is done by taking slices100units apart,ranging from our“start”of the highway x=0,to the x coordinate of the furthest vehicle on highway,rounded up to the nearest100.This last value,divided by100 we call N,since it corresponds to the number of slices on the highway for our calculation.
q=1
N X i=1...N Number of cars passing through x i
?
=q=
1
N X i=1...N100i?.
Since?is independent of the summand,we can factor it out.Additionally,we can re-express the sum using the fact that there are a?nite number of cars, labelled from1to M.So,
q=
1
N?X i=1...N X j=1...M bool(car j passes through x i)
Page4of13Control#29221 Where bool(A)is1if A is true and0if A is false.This may seem daunting, however,our computer simulation has the handy property of recording the?rst and last positions of every car.So,given any slice of highway x i,if a particular car starts before the slice at t=0,and ends up after the slice at t=?,then we know for a fact that it passed through the slice.This converts our rather ugly looking sum into the much more palatable form of
q=
1
100N?X j=1...M b x final x start c
where j runs over the indices of the cars.
Our simulation of one lane tra c uses random assignments in position and
velocity to accurately re-create tra c conditions.To account for the possible
discrepancy in car density due to this feature,we average car density over the
highway using a similar procedure to the one above.The space averaged density
k at a particular time is simply the number of cars on the highway behind the
furthest car and in front of the car furthest behind.So,the space averaged
density k is(#of cars)/(x most x least).However,the space averaged density varies in time,so to establish a proper relationship between q and k,we need to
time average k as well.This is done by averaging k over equal intervals of one
hundred seconds,so
k=1
6
600
X t=100#of cars at t
x most x least
where x most and x least are both functions of time,since the car in front will continue to move,as will the car in the back,and not necessarily at the same rates.For our single lane analysis,the car in front will remain in that position, whereas in multi-lane models further in our paper the car identity is not conserved. Additionally,in continuous tra c stream models x least can simply be replaced by0,since more cars will constantly be added during every time step near the start of the highway.
2.2Introduction to our Model
Here we will introduce a model for tra c in one lane.This is a very simple case as we won’t have to consider lane switching,each car on the road will only worry about the behavior of the car directly in front of it.Here is a?ow chart of the decisions:
Page5of13Control#29221
As a car in the system,your?rst choice is whether or not the follow distance you are currently maintaining is enough.We chose three car lengths to be the target follow distance,this is based on recommendations by driver’s manuals in the United States.We determine this by estimating both where we will be in one time step,and where the car in front of us will be.If this distance does not have the required spacing,we attempt to slow down until we achieve the safe spacing.If that spacing is achieved then the only other thing we,as a car,worry about is the speed limit.If we are below the speed limit,we accelerate,if we are at the speed limit then we continue on our journey.Note that in this model it is impossible to go above the speed limit as we only increase our velocity if we are below the speed limit,every other case maintains or loses velocity.With this model we can observe some of the statistics described above.
If tra c is light enough that any given car can safely reach the speed limit then we expect to see the?ow,q,increase as the density k increases until the amount of cars begins to a?ect the ability of drivers to safely reach the speed limit.After this point we expect to see a decline in q all the way to0,at which tra c is gridlocked.
As we can see in Figure1,the?ow rate,q,has a peak in the middle.This is the zone where tra c isn’t dense enough to a?ect tra c patterns.After a certain point this value quickly drops o?as the density of the highway approaches gridlock.This con?rms our analysis of the simple model and seems to agree with reality as far as we can tell.
Page6of13Control#29221
Figure1:Simulated results of tra c?ow vs density in single lane tra c
3Analysis of the Standard Maneuver
3.1Theoretical Approach
The standard maneuver is the rule stating that a driver may only use the left lane to pass someone,if they are not passing,then they should move back to the right lane.Intuitively this means that when there are a lot of lanes and a lot of tra c,the leftmost lanes will be underused and cause unnecessary congestion in the right lanes,this will be due to the fact that no one is using the left lanes for travel,but only to pass a slower car in front of themselves.Our initial thought was that this rule seemed very ine cient.In this model if a faster car approaches a slower car,if it is unable to immediately switch lanes to start passing,it will have to slow down.That slowing will propagate backward down the lane until a car is able to begin the maneuver.
3.2Computational Approach
In our computational approach to analyzing this rule,we made several changes to the basic model outlined in the previous section.We consider n lanes instead of only one,which introduces the possibility of cars switching lanes.This leads to a lengthy decision tree that must be gone through for each car in each time step.The general?ow is as follows:
Page7of13Control#29221
Unlike in the simple model,here we must consider an extra dimension,more lanes,and adherence to a new rule.As a car in the model,the?rst choice we will make is whether or not we want to pass the car in front of us.If they are moving at a lower velocity than us,we assume that we do want to pass them.If we want to pass them,then we?rst check if it is okay to merge left.If so,we can go ahead and change lanes,but if not we will assume that we cannot pass and according to the rule,we must attempt to get back into the right lane.So we check whether or not that is safe.If it is not,we simplify back to the case in the simple model where we only care about not getting too close to the car in front of us and maintaining the speed limit.
The expected behavior of this system is slightly di?erent than the simple model.In the case where there is low enough tra c density,we expect all tra c to be in the right lane and the system to behave like the one lane simple case. However,as we increase the density,instead of seeing a decrease in?ow where we would in the simple model,we expect to see?ow increase beyond this point because faster tra c will start to spill over into the left lanes until they can merge back.This will mean that the?ow can handle a denser system for longer. Eventually we will see a gridlock steady state when all of the lanes get?lled up, but that will take signi?cantly longer than in the simple model.
In Figure2we can see that the peak q value is higher than that in the simple model.This is because tra c is able to move more smoothly when merging is allowed.Tra c which would get stuck can choose to pass the obstruction and continue at a higher rate.We notice that the k value for which the system starts to decline is very similar to the simple model,meaning that it doesn’t handle a larger density of tra c than the simple model,but an equal amount,only more e ciently.
Page8of13Control#29221
Figure2:Simuated results for?ow vs density using“standard maneuver”rule 4Analysis of a New Rule
4.1Theoretical Approach
The motivation for this new rule was that driver’s inherently have a speed at which they are comfortable moving,if we group these drivers together,they can continue at that speed,this removes the e?ect of one slow down causing a ripple e?ect backwards down the lane.Faster tra c will move left,while slower tra c will move right.It is a very simple rule that drivers can easily understand.One potential downside of this model is that changing lanes either to the right or the left could become dangerous if the change in speed is drastically di?erent as a driver would have to either speed up or slow down in order to pass.
4.2Computational Approach
The?ow chart for our proposed rule is as follows:
Page9of13Control#29221
In this model each car has a target speed,either at,above,or below the speed limit.The further left a lane is,the higher average speed it has.Faster cars will want to move left and slower cars will want to move right.The decision in any time set for any given car starts by asking whether the car is in the optimal lane for its speed,in this case,the lane whose average speed is closest to the target speed.If they are,they use the behavior from the simple model.If they aren’t, then then will check and attempt to merge in the direction which changes their speed to be closer to their target speed.Given a section of tra c,we expect this model to have a fairly chaotic initial stage,then converge to a steady state behavior when each car is in it’s ideal lane.Overall we expect this to be more e cient in terms of tra c?ow than the standard maneuver because it will allow some tra c to move faster and will reduce the amount of slowing generated when a faster vehicle approaches a slower one and has to begin to slow down itself,which will then chain backwards to each car behind them for some depth of cars.In our case we use?ve lanes,the three left-most lanes are increments above the speed limit,the second from the right is at the speed limit,and the right lane is slightly below the speed limit.
Speci?cally we can expect that this rule will have much greater?ow than if we used the simple model in every lane of a multi-lane highway.In the case where we have multiple simple models the?ow will be limited by the speed limit and by any driver who drives below that.After the initial chaotic behavior of our new model,when every driver has found their ideal lane,we can expect a steady state where four of the lanes are moving at a rate faster than lanes of the simple model,and the?fth is moving slightly slower.Overall this translates to a higher q when density is high.
In Figure3we can see that the peak q value is higher and it occurs at a higher k value.This shows that the rule can handle a higher number of cars
Page10of13Control#29221
Figure3:Simulated results of tra c?ow vs density using new rule while still operating at a high rate.This con?rms what we would expect from this rule.The tra c merges a lot in the?rst time steps,then it settles into a highly e cient pattern where more lanes are able to move quicker.
5Modi?cations and Extensions
5.1Driving on the Left Side
Our model is not dependent on orientation.If we simply?ip the orientation of the drivers and the roads we get an identical problem,di?erent only in direction.
5.2Safety Trade-o?
Of course,a natural concern for any heuristic about driving is safety.According to the World Health Organization,road tra c injuries caused an estimated1.24 million deaths worldwide in the year2010.Our models take into account the potential for automobiles to hit each other.On a?ve lane highway with a speed limit of71miles per hour,due to limitations of our model,we experience a small amount of collisions immediately,but this number quickly goes to zero when we reach a steady state.In all of the tra c rules that we modeled,collisions were demonstrated to occur at a higher incidence when more complicated behavior such as changing lanes,or accelerating more aggressively was prevalent.This is to be expected,compared to the much simpler case of several drivers driving in a single lane,where intuitively,we would expect that collisions are more easily preventable.Our models re?ect this by producing less car collisions in the simple single lane situation,than are produced in more complicated multi-lane situation.
Page11of13Control#29221
5.3Varied Speed Limits
When we run our models with higher than average speed limits,we notice a dramatic increase in collisions.This is to be expected,as an increase in speed leads to more di culty slowing down in the event of a change in velocity of the ?ow.Cars can only brake at a certain rate,if we go fast enough that this doesn’t signi?cantly impact the distance we will travel in the seconds before a collision, then collisions become very hard to avoid.This is made even worse in models where cars actively switch lanes,the combination of high speeds and the fact that our model has limited ability to predict the safety of a merge gives us a frightening fatality rate for even a small number of drivers.However there are certain systems where extremely high speeds would only improve the?ow. 5.4An Ideal System
In an ideal system the whole tra c system is controlled by an intelligent system. Under this assumption we can have cars moving at a much faster speed and have safety be una?ected.Imagine a road where every car was controlled by a central computer.This computer knows the position and velocity of each car and can adjust the acceleration of any car.The biggest safety hazard on highways is when people travel at di?erent speeds,this can be removed from the equation when a computer can have each car in the system travel at precisely the same speed.In a line of cars all moving at the same rate,there is no chance of a collision.
Taking this system a step further we can imagine that to handle merging the computer could slow down portions of tra c by a small,fractional amount, in order to create gaps for more cars to merge into the system.When done by a centralized power like this,reaction,overreaction,and any propagation of slowing can be minimized.The e?ect of propagation can be absorbed in smaller and smaller amounts until it is unnoticeable to a human observer.Under these conditions we can reach what would be a theoretical maximal tra c?ow,limited locally only by the top speed of the slowest vehicle and globally by the top speed of a car.
In such a system the?ow q is maximized as there is no slow down when adding more cars,the curve of k vs.q will have a plateaued maximum because once we reach saturation,adding more cars won’t increase the?ow,but it won’t decrease it either.The only limit on q is on how perfect the control is and the physical capacities of cars.
6Conclusion
In this paper we examined a discrete model for modeling tra c patterns based on individual decisions of drivers.This is a much di?erent problem than examining the system from a macroscopic point of view,however both methods are useful for examining di?erent properties.We chose to focus on the microscopic system because we are better able to see how rules a?ect individual drivers and can see
Page12of13Control#29221 trends on tra c as a whole.We compared di?erent rules to the base case of a simple model where cars don’t switch lanes and only worry about following the speed limit,and not hitting the car in front of them.
The?rst rule we analyzed stated that a driver should remain in the right lane unless they are passing another slower car.We called this the standard model.We found that this was more e?ective than the base case.Faster tra c was able to escape a slower?ow and spill into additional lanes when it needed to,which allowed for a reduction in the amount of slowing observed when the road started to become crowded.
The second rule was our proposed rule.This rule dictated that faster cars move to the left and slower cars move to the right.This creates an ideal lane for each driver based on the speed they want to travel.After the system settles and each driver?nds their preferred lane,the?ow is much improved over both the simple and standard model.This was due to the fact that in the steady state more lanes were moving faster than in the simple or standard case.Faster tra c was not impeded by slower tra c,which increased the overall?ow at high densities.In the steady state,this model is also safer.Tra c is at its safest when every vehicle is traveling at the same speed,this reduces the need for reaction due to a driver having to slow down for tra c and it is impossible to run into another driver unless you are switching lanes incorrectly or there is an unforeseen accident which requires a change in speed.In each of the other models we have tra c which wants to move faster,but cannot due to a slower car.They are then required to slow down,which puts them and everyone behind them at risk because it requires action on behalf of other drivers.
We found that our model is independent of the side of the road it is standard to drive on;simply changing the orientation is a su cient modi?cation.If we consider an ideal system,we can imagine that it is an improved version of our proposed rule,where every lane is as fast the vehicles can safely handle,and merging is no longer a threat to safety because it can be precisely and centrally controlled.
We can think of several extensions and improvements to our model,the ?rst being to increase the scale and accuracy.We were limited in our time and hardware,but ideally we would run a longer simulation with many more cars and remove most of the simplifying assumptions outlined earlier.This would give us a better idea of the dynamics of each scenario.It is also possible to extend the discussion to a continuous system governed by partial di?erential equations,this would give a clearer picture of large scale phenomena,but is poor at describing the behavior of individual drivers.There are even models in the current literature that combine the macroscopic and microscopic scales into a mesoscopic model. These are very involved models,and are similar to computing gas dynamics by the interactions of individual particles.Given adequate resources,these types of models could be very informative and provide much more in-depth and accurate results than either the macro-or micro-scopes could.
Page13of13Control#29221
7References
[1]Ding Ding,Modeling and simulation of highway traf-
?c using a cellular automaton approach https://www.360docs.net/doc/e77469106.html,/smash/get/diva2:483914/FULLTEXT01.pdf,2011.
[2]Serge Hoogendoorn and Victor Knoop,Tra c?ow theory and modelling
http://www.victorknoop.eu/research/papers/chapter vanwee.pdf,2012. [3]Fred L.Hall,Tra c Stream Characteristics
https://https://www.360docs.net/doc/e77469106.html,/publications/research/operations/tft/chap2.pdf, 1992.
[4]M.J.Lighthill and G.B.Whitham On kinematic waves II,A theory of tra c
?ow on long crowded roads1955
09年美赛A题优秀论文翻译
A题设计一个交通环岛 在许多城市和社区都建立有交通环岛,既有多条行车道的大型环岛(例如巴黎的凯旋门和曼谷的胜利纪念碑路口),又有一至两条行车道的小型环岛。有些环岛在进入口设有“停车”标志或者让行标志,其目的是给已驶入环岛的车辆提供行车优先权;而在一些环岛的进入口的逆向一侧设立的让行标志是为了向即将驶入环岛的车辆提供行车优先权;还有一些环岛会在入口处设立交通灯(红灯会禁止车辆右转);也可能会有其他的设计方案。 这一设计的目的在于利用一个模型来决定如何最优地控制环岛内部,周围以及外部的交通流。该设计的目的在于可利用模型做出最佳的方案选择以及分析影响选择的众多因素。解决方案中需要包括一个不超过两页纸,双倍行距打印的技术摘要,它可以指导交通工程师利用你们模型对任何特殊的环岛进行适当的流量控制。该模型可以总结出在何种情况之下运用哪一种交通控制法为最优。当考虑使用红绿灯的时候,给出一个绿灯的时长的控制方法(根据每日具体时间以及其他因素进行协调)。找一些特殊案例,展示你的模型的实用性。 标题:一个环来控制一切:优化交通圈。 安德里亚?利维亚伦 安德烈娅?利维 拉塞尔?梅里克 哈维姆德学院 顾问:苏珊 摘要 我们的目的是利用车辆动力学考虑在圆形交叉路口的道路情况。我们主要根据进入圆形道路的速度决定最好的方式来控制车流量。我们假设在一个车道通过圆形道路循环,这样交通输入量能够被调节。(也就是,不会有优先的交通输入量) 对于我们的模型,可改变的参数是排队等候进入的速率,进入圆形道路的速率(服务速率),这个圆形道路最大的容量和离开这个道路的速率。我们使用带有队列和交通圈的隔室模型作为隔间。来自外界的车辆首先进行排队等候,然后进入圆环交叉路口,最后离开到外界。我们把服务速率和离开速率作为在圆环交叉路口的车辆数量参考。 另外,我们利用计算机来拟态一个可见表示,发生在不同情形下的圆环交叉路口。允许我们检验不同的情况,例如不平等的交通流量由于不同的队列,一些十字路口比其他车辆有一个更高的概率。这个拟态模仿实施栩栩如生,例如如何当前面是空道路时进行加速,而当前面有其他车辆时进行减速。大多数情况下,我们发现:一个高服务效率能够保持交通顺畅的最佳方式,这意味着对于进入交通的效率是最有效的。然而,当交通变得拥堵时,较低的服务率更好的适应了交通,这指示应该使用一个红绿灯。所以,在不同时间段,依靠预测中的交通流量,一个信号灯应该被安装进行循环实现。
数学建模美赛o奖论文
For office use only T1________________ T2________________ T3________________ T4________________ Team Control Number 55069 Problem Chosen A For office use only F1________________ F2________________ F3________________ F4________________ 2017 MCM/ICM Summary Sheet The Rehabilitation of the Kariba Dam Recently, the Institute of Risk Management of South Africa has just warned that the Kariba dam is in desperate need of rehabilitation, otherwise the whole dam would collapse, putting 3.5 million people at risk. Aimed to look for the best strategy with the three options listed to maintain the dam, we employ AHP model to filter factors and determine two most influential criteria, including potential costs and benefits. With the weight of each criterion worked out, our model demonstrates that option 3is the optimal choice. According to our choice, we are required to offer the recommendation as to the number and placement of the new dams. Regarding it as a set covering problem, we develop a multi-objective optimization model to minimize the number of smaller dams while improving the water resources management capacity. Applying TOPSIS evaluation method to get the demand of the electricity and water, we solve this problem with genetic algorithm and get an approximate optimal solution with 12 smaller dams and determine the location of them. Taking the strategy for modulating the water flow into account, we construct a joint operation of dam system to simulate the relationship among the smaller dams with genetic algorithm approach. We define four kinds of year based on the Kariba’s climate data of climate, namely, normal flow year, low flow year, high flow year and differential year. Finally, these statistics could help us simulate the water flow of each month in one year, then we obtain the water resources planning and modulating strategy. The sensitivity analysis of our model has pointed out that small alteration in our constraints (including removing an important city of the countries and changing the measurement of the economic development index etc.) affects the location of some of our dams slightly while the number of dams remains the same. Also we find that the output coefficient is not an important factor for joint operation of the dam system, for the reason that the discharge index and the capacity index would not change a lot with the output coefficient changing.
美赛论文要点
摘要: 第一段:写论文解决什么问题 1.问题的重述 a. 介绍重点词开头: 例1:“Hand move” irrigation, a cheap but labor-intensive system used on small farms, consists of a movable pipe with sprinkler on top that can be attached to a stationary main. 例2:……is a real-life common phenomenon with many complexities. 例3:An (effective plan) is crucial to……… b. 直接指出问题: 例 1:We find the optimal number of tollbooths in a highway toll-plaza for a given number of highway lanes: the number of tollbooths that minimizes average delay experienced by cars. 例2:A brand-new university needs to balance the cost of information technology security measures with the potential cost of attacks on its systems. 例3:We determine the number of sprinklers to use by analyzing the energy and motion of water in the pipe and examining the engineering parameters of sprinklers available in the market. 例4: After mathematically analyzing the …… problem, our modeling group would like to pres ent our conclusions, strategies, (and recommendations )to the ……. 例5:Our goal is... that (minimizes the time )………. 2.解决这个问题的伟大意义 反面说明。如果没有…… Without implementing defensive measure, the university is exposed to an expected loss of $8.9 million per year. 3.总的解决概述 a.通过什么方法解决什么问题 例:We address the problem of optimizing amusement park enjoyment through distributing Quick Passes (QP), reservation slips that ideally allow an individual to spend less time waiting in line. b.实际问题转化为数学模型
数学建模国赛一等奖论文
电力市场输电阻塞管理模型 摘要 本文通过设计合理的阻塞费用计算规则,建立了电力市场的输电阻塞管理模型。 通过对各机组出力方案实验数据的分析,用最小二乘法进行拟合,得到了各线路上有功潮流关于各发电机组出力的近似表达式。按照电力市场规则,确定各机组的出力分配预案。如果执行该预案会发生输电阻塞,则调整方案,并对引起的部分序内容量和序外容量的收益损失,设计了阻塞费用计算规则。 通过引入危险因子来反映输电线路的安全性,根据安全且经济的原则,把输电阻塞管理问题归结为:以求解阻塞费用和危险因子最小值为目标的双目标规划问题。采用“两步走”的策略,把双目标规划转化为两次单目标规划:首先以危险因子为目标函数,得到其最小值;然后以其最小值为约束,找出使阻塞管理费用最小的机组出力分配方案。 当预报负荷为982.4MW时,分配预案的清算价为303元/MWh,购电成本为74416.8元,此时发生输电阻塞,经过调整后可以消除,阻塞费用为3264元。 当预报负荷为1052.8MW时,分配预案的清算价为356元/MWh,购电成本为93699.2元,此时发生输电阻塞,经过调整后可以使用线路的安全裕度输电,阻塞费用为1437.5元。 最后,本文分析了各线路的潮流限值调整对最大负荷的影响,据此给电网公司提出了建议;并提出了模型的改进方案。
一、问题的重述 我国电力系统的市场化改革正在积极、稳步地进行,随着用电紧张的缓解,电力市场化将进入新一轮的发展,这给有关产业和研究部门带来了可预期的机遇和挑战。 电网公司在组织电力的交易、调度和配送时,必须遵循电网“安全第一”的原则,同时按照购电费用最小的经济目标,制订如下电力市场交易规则: 1、以15分钟为一个时段组织交易,每台机组在当前时段开始时刻前给出下一个时段的报价。各机组将可用出力由低到高分成至多10段报价,每个段的长度称为段容量,每个段容量报一个段价,段价按段序数单调不减。 2、在当前时段内,市场交易-调度中心根据下一个时段的负荷预报、每台机组的报价、当前出力和出力改变速率,按段价从低到高选取各机组的段容量或其部分,直到它们之和等于预报的负荷,这时每个机组被选入的段容量或其部分之和形成该时段该机组的出力分配预案。最后一个被选入的段价称为该时段的清算价,该时段全部机组的所有出力均按清算价结算。 电网上的每条线路上有功潮流的绝对值有一安全限值,限值还具有一定的相对安全裕度。如果各机组出力分配方案使某条线路上的有功潮流的绝对值超出限值,称为输电阻塞。当发生输电阻塞时,需要按照以下原则进行调整: 1、调整各机组出力分配方案使得输电阻塞消除; 2、如果1做不到,可以使用线路的安全裕度输电,以避免拉闸限电,但要使每条 线路上潮流的绝对值超过限值的百分比尽量小; 3、如果无论怎样分配机组出力都无法使每条线路上的潮流绝对值超过限值的百分 比小于相对安全裕度,则必须在用电侧拉闸限电。 调整分配预案后,一些通过竞价取得发电权的发电容量不能出力;而一些在竞价中未取得发电权的发电容量要在低于对应报价的清算价上出力。因此,发电商和网方将产生经济利益冲突。网方应该为因输电阻塞而不能执行初始交易结果付出代价,网方在结算时应该适当地给发电商以经济补偿,由此引起的费用称之为阻塞费用。网方在电网安全运行的保证下应当同时考虑尽量减少阻塞费用。 现在需要完成的工作如下: 1、某电网有8台发电机组,6条主要线路,附件1中表1和表2的方案0给出了各机组的当前出力和各线路上对应的有功潮流值,方案1~32给出了围绕方案0的一些实验数据,试用这些数据确定各线路上有功潮流关于各发电机组出力的近似表达式。 2、设计一种简明、合理的阻塞费用计算规则,除考虑电力市场规则外,还需注意:在输电阻塞发生时公平地对待序内容量不能出力的部分和报价高于清算价的序外容量出力的部分。 3、假设下一个时段预报的负荷需求是982.4MW,附件1中的表3、表4和表5分别给出了各机组的段容量、段价和爬坡速率的数据,试按照电力市场规则给出下一个时段各机组的出力分配预案。 4、按照表6给出的潮流限值,检查得到的出力分配预案是否会引起输电阻塞,并在发生输电阻塞时,根据安全且经济的原则,调整各机组出力分配方案,并给出与该方案相应的阻塞费用。 5、假设下一个时段预报的负荷需求是1052.8MW,重复3~4的工作。 二、问题的分析
美赛论文模板(强烈推荐)
Titile Summary During cell division, mitotic spindles are assembled by microtubule-based motor proteins1, 2. The bipolar organization of spindles is essential for proper segregation of chromosomes, and requires plus-end-directed homotetrameric motor proteins of the widely conserved kinesin-5 (BimC) family3. Hypotheses for bipolar spindle formation include the 'push?pull mitotic muscle' model, in which kinesin-5 and opposing motor proteins act between overlapping microtubules2, 4, 5. However, the precise roles of kinesin-5 during this process are unknown. Here we show that the vertebrate kinesin-5 Eg5 drives the sliding of microtubules depending on their relative orientation. We found in controlled in vitro assays that Eg5 has the remarkable capability of simultaneously moving at 20 nm s-1 towards the plus-ends of each of the two microtubules it crosslinks. For anti-parallel microtubules, this results in relative sliding at 40 nm s-1, comparable to spindle pole separation rates in vivo6. Furthermore, we found that Eg5 can tether microtubule plus-ends, suggesting an additional microtubule-binding mode for Eg5. Our results demonstrate how members of the kinesin-5 family are likely to function in mitosis, pushing apart interpolar microtubules as well as recruiting microtubules into bundles that are subsequently polarized by relative sliding. We anticipate our assay to be a starting point for more sophisticated in vitro models of mitotic spindles. For example, the individual and combined action of multiple mitotic motors could be tested, including minus-end-directed motors opposing Eg5 motility. Furthermore, Eg5 inhibition is a major target of anti-cancer drug development, and a well-defined and quantitative assay for motor function will be relevant for such developments
全国数模竞赛优秀论文
一、基础知识 1.1 常见数学函数 如:输入x=[-4.85 -2.3 -0.2 1.3 4.56 6.75],则: ceil(x)= -4 -2 0 2 5 7 fix(x) = -4 -2 0 1 4 6 floor(x) = -5 -3 -1 1 4 6 round(x) = -5 -2 0 1 5 7 1.2 系统的在线帮助 1 help 命令: 1.当不知系统有何帮助内容时,可直接输入help以寻求帮助: >>help(回车) 2.当想了解某一主题的内容时,如输入: >> help syntax(了解Matlab的语法规定) 3.当想了解某一具体的函数或命令的帮助信息时,如输入: >> help sqrt (了解函数sqrt的相关信息)
2 lookfor命令 现需要完成某一具体操作,不知有何命令或函数可以完成,如输入: >> lookfor line (查找与直线、线性问题有关的函数) 1.3 常量与变量 系统的变量命名规则:变量名区分字母大小写;变量名必须以字母打头,其后可以是任意字母,数字,或下划线的组合。此外,系统内部预先定义了几个有特殊意 1 数值型向量(矩阵)的输入 1.任何矩阵(向量),可以直接按行方式 ...输入每个元素:同一行中的元素用逗号(,)或者用空格符来分隔;行与行之间用分号(;)分隔。所有元素处于一方括号([ ])内; 例1: >> Time = [11 12 1 2 3 4 5 6 7 8 9 10] >> X_Data = [2.32 3.43;4.37 5.98] 2 上面函数的具体用法,可以用帮助命令help得到。如:meshgrid(x,y) 输入x=[1 2 3 4]; y=[1 0 5]; [X,Y]=meshgrid(x, y),则 X = Y =
2014年数学建模美赛题目原文及翻译
2014年数学建模美赛题目原文及翻译 作者:Ternence Zhang 转载注明出处:https://www.360docs.net/doc/e77469106.html,/zhangtengyuan23 MCM原题PDF: https://www.360docs.net/doc/e77469106.html,/detail/zhangty0223/6901271 PROBLEM A: The Keep-Right-Except-To-Pass Rule In countries where driving automobiles on the right is the rule (that is, USA, China and most other countries except for Great Britain, Australia, and some former British colonies), multi-lane freeways often employ a rule that requires drivers to drive in the right-most lane unless they are passing another vehicle, in which case they move one lane to the left, pass, and return to their former travel lane. Build and analyze a mathematical model to analyze the performance of this rule in light and heavy traffic. You may wish to examine tradeoffs between traffic flow and safety, the role of under- or over-posted speed limits (that is, speed limits that are too low or too high), and/or other factors that may not be
SARS传播的数学模型 数学建模全国赛优秀论文
SARS传播的数学模型 (轩辕杨杰整理) 摘要 本文分析了题目所提供的早期SARS传播模型的合理性与实用性,认为该模型可以预测疫情发展的大致趋势,但是存在一定的不足.第一,混淆了累计患病人数与累计确诊人数的概念;第二,借助其他地区数据进行预测,后期预测结果不够准确;第三,模型的参数L、K的设定缺乏依据,具有一定的主观性. 针对早期模型的不足,在系统分析了SARS的传播机理后,把SARS的传播过程划分为:征兆期,爆发期,高峰期和衰退期4个阶段.将每个阶段影响SARS 传播的因素参数化,在传染病SIR模型的基础上,改进得到SARS传播模型.采用离散化的方法对本模型求数值解得到:北京SARS疫情的预测持续时间为106天,预测SARS患者累计2514人,与实际情况比较吻合. 应用SARS传播模型,对隔离时间及隔离措施强度的效果进行分析,得出结论:“早发现,早隔离”能有效减少累计患病人数;“严格隔离”能有效缩短疫情持续时间. 在建立模型的过程中发现,需要认清SARS传播机理,获得真实有效的数据.而题目所提供的累计确诊人数并不等于同期累计患病人数,这给模型的建立带来不小的困难. 本文分析了海外来京旅游人数受SARS的影响,建立时间序列半参数回归模型进行了预测,估算出SARS会对北京入境旅游业造成23.22亿元人民币损失,并预计北京海外旅游人数在10月以前能恢复正常. 最后给当地报刊写了一篇短文,介绍了建立传染病数学模型的重要性.
1.问题的重述 SARS (严重急性呼吸道综合症,俗称:非典型肺炎)的爆发和蔓延使我们认识到,定量地研究传染病的传播规律,为预测和控制传染病蔓延创造条件,具有很高的重要性.现需要做以下工作: (1) 对题目提供的一个早期模型,评价其合理性和实用性. (2) 建立自己的模型,说明优于早期模型的原因;说明怎样才能建立一个真正能够预测以及能为预防和控制提供可靠、足够信息的模型,并指出这样做的困难;评价卫生部门采取的措施,如:提前和延后5天采取严格的隔离措施,估计对疫情传播的影响. (3) 根据题目提供的数据建立相应的数学模型,预测SARS 对社会经济的影响. (4) 给当地报刊写一篇通俗短文,说明建立传染病数学模型的重要性. 2.早期模型的分析与评价 题目要求建立SARS 的传播模型,整个工作的关键是建立真正能够预测以及能为预防和控制提供可靠、足够的信息的模型.如何结合可靠、足够这两个要求评价一个模型的合理性和实用性,首先需要明确: 合理性定义 要求模型的建立有根据,预测结果切合实际. 实用性定义 要求模型能全面模拟真实情况,以量化指标指导实际. 所以合理的模型能为预防和控制提供可靠的信息;实用的模型能为预防和控制提供足够的信息. 2.1早期模型简述 早期模型是一个SARS 疫情分析及疫情走势预测的模型, 该模型假定初始时刻的病例数为0N , 平均每病人每天可传染K 个人(K 一般为小数),K 代表某种社会环境下一个病人传染他人的平均概率,与全社会的警觉程度、政府和公众采取的各种措施有关.整个模型的K 值从开始到高峰期间保持不变,高峰期后 10天的范围内K 值逐步被调整到比较小的值,然后又保持不变. 平均每个病人可以直接感染他人的时间为L 天.整个模型的L 一直被定为20.则在L 天之内,病例数目的增长随时间t (单位天)的关系是: t k N t N )1()(0+?= 考虑传染期限L 的作用后,变化将显著偏离指数律,增长速度会放慢.采用半模拟循环计算的办法,把到达L 天的病例从可以引发直接传染的基数中去掉. 2.2早期模型合理性评价 根据早期模型对北京疫情的分析与预测,其先将北京的病例起点定在3月1日,经过大约59天在4月29日左右达到高峰,然后通过拟合起点和4月20日以后的数据定出高峰期以前的K =0.13913.高峰期后的K 值按香港情况变化,即10天范围内K 值逐步被调整到0.0273.L 恒为20.由此画出北京3月1日至5月7日疫情发展趋势拟合图像以及5月7日以后的疫情发展趋势预测图像,如图1.
数学建模美赛2012MCM B论文
Camping along the Big Long River Summary In this paper, the problem that allows more parties entering recreation system is investigated. In order to let park managers have better arrangements on camping for parties, the problem is divided into four sections to consider. The first section is the description of the process for single-party's rafting. That is, formulating a Status Transfer Equation of a party based on the state of the arriving time at any campsite. Furthermore, we analyze the encounter situations between two parties. Next we build up a simulation model according to the analysis above. Setting that there are recreation sites though the river, count the encounter times when a new party enters this recreation system, and judge whether there exists campsites available for them to station. If the times of encounter between parties are small and the campsite is available, the managers give them a good schedule and permit their rafting, or else, putting off the small interval time t until the party satisfies the conditions. Then solve the problem by the method of computer simulation. We imitate the whole process of rafting for every party, and obtain different numbers of parties, every party's schedule arrangement, travelling time, numbers of every campsite's usage, ratio of these two kinds of rafting boats, and time intervals between two parties' starting time under various numbers of campsites after several times of simulation. Hence, explore the changing law between the numbers of parties (X) and the numbers of campsites (Y) that X ascends rapidly in the first period followed by Y's increasing and the curve tends to be steady and finally looks like a S curve. In the end of our paper, we make sensitive analysis by changing parameters of simulation and evaluate the strengths and weaknesses of our model, and write a memo to river managers on the arrangements of rafting. Key words: Camping;Computer Simulation; Status Transfer Equation
(完整)美赛一等奖经验总结,推荐文档
当我谈数学建模时我谈些什么——美赛一等奖经验总结 作者:彭子未 前言:2012 年3月28号晚,我知道了美赛成绩,一等奖(Meritorus Winner),没有太多的喜悦,只是感觉释怀,一年以来的努力总算有了回报。从国赛遗憾丢掉国奖,到美赛一等,这一路走来太多的不易,感谢我的家人、队友以及朋友的支持,没有你们,我无以为继。 这篇文章在美赛结束后就已经写好了,算是对自己建模心得体会的一个总结。现在成绩尘埃落定,我也有足够的自信把它贴出来,希望能够帮到各位对数模感兴趣的同学。 欢迎大家批评指正,欢迎与我交流,这样我们才都能进步。 个人背景:我2010年入学,所在的学校是广东省一所普通大学,今年大二,学工商管理专业,没学过编程。 学校组织参加过几届美赛,之前唯一的一个一等奖是三年前拿到的,那一队的主力师兄凭借这一奖项去了北卡罗来纳大学教堂山分校,学运筹学。今年再次拿到一等奖,我创了两个校记录:一是第一个在大二拿到数模美赛一等奖,二是第一个在文科专业拿数模美赛一等奖。我的数模历程如下: 2011.4 校内赛三等奖 2011.8 通过选拔参加暑期国赛培训(学校之前不允许大一学生参加) 2011.9 国赛广东省二等奖 2011.11 电工杯三等奖 2012.2 美赛一等奖(Meritorious Winner) 动机:我参加数学建模的动机比较单纯,完全是出于兴趣。我的专业是工商管理,没有学过编程,觉得没必要学。我所感兴趣的是模型本身,它的思想,它的内涵,它的发展过程、它的适用问题等等。我希望通过学习模型,能够更好的去理解一些现象,了解其中蕴含的数学机理。数学模型中包含着一种简洁的哲学,深刻而迷人。 当然获得荣誉方面的动机可定也有,谁不想拿奖呢? 模型:数学模型的功能大致有三种:评价、优化、预测。几乎所有模型都是围绕这三种功能来做的。比如,今年美赛A题树叶分类属于评价模型,B题漂流露营安排则属于优化模型。 对于不同功能的模型有不同的方法,例如评价模型方法有层次分析、模糊综合评价、熵值法等;优化模型方法有启发式算法(模拟退火、遗传算法等)、仿真方法(蒙特卡洛、元
数学建模全国赛07年A题一等奖论文
关于中国人口增长趋势的研究 【摘要】 本文从中国的实际情况和人口增长的特点出发,针对中国未来人口的老龄化、出生人口性别比以及乡村人口城镇化等,提出了Logistic、灰色预测、动态模拟等方法进行建模预测。 首先,本文建立了Logistic阻滞增长模型,在最简单的假设下,依照中国人口的历史数据,运用线形最小二乘法对其进行拟合,对2007至2020年的人口数目进行了预测,得出在2015年时,中国人口有13.59亿。在此模型中,由于并没有考虑人口的年龄、出生人数男女比例等因素,只是粗略的进行了预测,所以只对中短期人口做了预测,理论上很好,实用性不强,有一定的局限性。 然后,为了减少人口的出生和死亡这些随机事件对预测的影响,本文建立了GM(1,1) 灰色预测模型,对2007至2050年的人口数目进行了预测,同时还用1990至2005年的人口数据对模型进行了误差检验,结果表明,此模型的精度较高,适合中长期的预测,得出2030年时,中国人口有14.135亿。与阻滞增长模型相同,本模型也没有考虑年龄一类的因素,只是做出了人口总数的预测,没有进一步深入。 为了对人口结构、男女比例、人口老龄化等作深入研究,本文利用动态模拟的方法建立模型三,并对数据作了如下处理:取平均消除异常值、对死亡率拟合、求出2001年市镇乡男女各年龄人口数目、城镇化水平拟合。在此基础上,预测出人口的峰值,适婚年龄的男女数量的差值,人口老龄化程度,城镇化水平,人口抚养比以及我国“人口红利”时期。在模型求解的过程中,还对政府部门提出了一些有针对性的建议。此模型可以对未来人口做出细致的预测,但是需要处理的数据量较大,并且对初始数据的准确性要求较高。接着,我们对对模型三进行了改进,考虑人为因素的作用,加入控制因子,使得所预测的结果更具有实际意义。 在灵敏度分析中,首先针对死亡率发展因子θ进行了灵敏度分析,发现人口数量对于θ的灵敏度并不高,然后对男女出生比例进行灵敏度分析得出其灵敏度系数为0.8850,最后对妇女生育率进行了灵敏度分析,发现在生育率在由低到高的变化过程中,其灵敏度在不断增大。 最后,本文对模型进行了评价,特别指出了各个模型的优缺点,同时也对模型进行了合理性分析,针对我国的人口情况给政府提出了建议。 关键字:Logistic模型灰色预测动态模拟 Compertz函数
国赛优秀论文
B甲004 目录 摘要 (3) 关键词 (3) 一、系统方案 (3) 1.1、方案比较与论证 (3) 1.1.1、控制器模块 (3) 1.1.2、电机及驱动模块 (3) 1.1.3、测速模块 (4) 1.1.4、音频产生模块 (4) 1.1.5、无线收发模块 (4) 1.1.6、声音采集处理模块 (4) 1.2、最终方案 (4) 二、电路设计 (5) 2.1、系统组成 (5) 2.2、电动机驱动电路 (5) 2.3、行程测量模块 (5) 2.4、声光报警模块 (6) 2.5、周期性音频脉冲信号产生模块 (6) 2.6、无线收发模块设计 (6) 2.7、声音采集计算系统 (6) 三、软件设计 (7) 3.1、电机驱动部分流程图 (7) 3.2、主程序流程图 (7) 3.3单片机控制MMC-1芯片的程序 (7) 3.4无线接收模块程序 (7) 四、系统测试 (8) 4.1、测试仪器 (8) 4.2、调试 (8) 4.2.1 速度调试 (8) 4.2.2 功率放大测试 (8) 4.2.3 声源频率测试 (8) 4.2.4 声音接收测试 (8) 五、总结 (9) 5.1、结论 (9) 5.2、结束语 (9) 六、参考文献 (9) 七、附录 (9) 附录一、部分电路原理图 (9) 附录二、主程序流程图 (11) 附录三、部分程序附录 (13)
摘要: 本课题设计制作小组本着简单、准确、可靠、稳定、通用、性价比低的原则,采用STC89C52作为声源系统的控制核心,使用凌阳SPCE061A作为音频信号分析处理系统核心,应用电机控制ASSP芯片MMC-1驱动电机。本系统电路分为声源移动模块,声音产生模块,声音采集处理模块,无线控制模块和显示报警模块。声音收发和无线传输模块测量声源与声音接收器之间的距离,控制声源移动。首先测量声源S距A、B的距离差,距离差为零表示小车已运动到OX线,然后测量S距A、C的距离差,距离差为零表示小车寻找到W点。小车在OX线上运动时,利用S距A、B的距离差校正路线,同时声光报警,LCD液晶显示屏显示小车运行路程和时间。 关键词:STC89C52;电机控制芯片MMC-1;PT2262/2272无线收发;周期性音频脉冲信号;TEA2025B音频放大 一、系统方案 1.1方案比较与论证 根据题目要求,本系统主要由控制器模块、直流电机及其驱动模块、声音产生模块,声音采集处理模块和无线控制模块、声光报警模块等构成。为较好的实现各模块的功能,我们分别设计了几种方案并分别进行了论证。 1.1.1控制器模块 方案一:采用大规模可编程逻辑器件(如FPGA)作为系统的控制中心,目前,大规模可编程逻辑器件容量不断增大,速度不断提高,且多具有ISP功能,也可以在不改变硬件电路的情况下改变功能,但在本系统中,它的高处理功能得不到从分利用,还考虑到VHDL语言描述也没有单片机语言那么方便,所以这个方案不采用。 方案二:采用单片机STC89C52作为中心控制器。STC89C52单片机算数运算功能强,软件编程灵活,自由度大,具有超低功耗,抗干扰能力强等特点。还具有ISP在线编程功能,在改写单片机存储内部的程序时不需要将单片机从工作环境中取出,方便快捷。在后来的实验中我们发现,STC89C52精确度和运算速度也都完全符合我们系统的要求。故采用STC89C52单片机为我们整个系统的控制核心。 1.1.2 电机及驱动模块 采用电机控制ASSP 芯片MMC-1驱动(实物图如图1)。MMC-1为多通道两相四线式步进电机/直流电机控制芯片,基于NEC 电子16 位通用MCU( PD78F1203)固化专用程序实现,支持UART 和SPI 串行接口。MMC-1 共有三个通道电机控制单元,通过设置寄存器可分别设置工作模式,实现不同功能。可以用来驱动直流电机和步进电机。 方案一:采用步进电机。步进电机是数字控制电机,不但控制精度高,而且简单可靠,但价格过高,重量大,占用端口资源多且控制复杂,不予采用。 2
美赛数学建模比赛论文模板
The Keep-Right-Except-To-Pass Rule Summary As for the first question, it provides a traffic rule of keep right except to pass, requiring us to verify its effectiveness. Firstly, we define one kind of traffic rule different from the rule of the keep right in order to solve the problem clearly; then, we build a Cellular automaton model and a Nasch model by collecting massive data; next, we make full use of the numerical simulation according to several influence factors of traffic flow; At last, by lots of analysis of graph we obtain, we indicate a conclusion as follow: when vehicle density is lower than 0.15, the rule of lane speed control is more effective in terms of the factor of safe in the light traffic; when vehicle density is greater than 0.15, so the rule of keep right except passing is more effective In the heavy traffic. As for the second question, it requires us to testify that whether the conclusion we obtain in the first question is the same apply to the keep left rule. First of all, we build a stochastic multi-lane traffic model; from the view of the vehicle flow stress, we propose that the probability of moving to the right is 0.7and to the left otherwise by making full use of the Bernoulli process from the view of the ping-pong effect, the conclusion is that the choice of the changing lane is random. On the whole, the fundamental reason is the formation of the driving habit, so the conclusion is effective under the rule of keep left. As for the third question, it requires us to demonstrate the effectiveness of the result advised in the first question under the intelligent vehicle control system. Firstly, taking the speed limits into consideration, we build a microscopic traffic simulator model for traffic simulation purposes. Then, we implement a METANET model for prediction state with the use of the MPC traffic controller. Afterwards, we certify that the dynamic speed control measure can improve the traffic flow . Lastly neglecting the safe factor, combining the rule of keep right with the rule of dynamical speed control is the best solution to accelerate the traffic flow overall. Key words:Cellular automaton model Bernoulli process Microscopic traffic simulator model The MPC traffic control